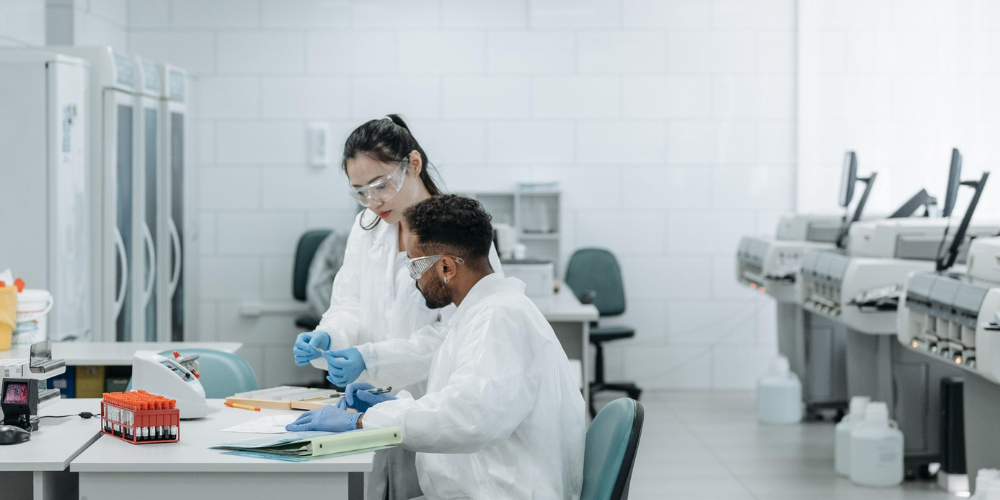
Ying Jiang, PhD, is Senior Director, Biostatistics of Keros Therapeutics.
How did you get into statistics and biometrics in biotech?
I went to graduate school for biology initially. When I first joined the industry as a biostatistician with Allergan 14 years ago, there was a sizable statistical group with around 30 people. It was both comforting and intimidating, because everyone spoke the same statistical language. I didn’t realize how much I benefited from my biology background until much later. It helped me ask better questions and communicate the statistics with my cross functional colleagues.
How can CMOs better communicate with their statisticians?
Treat them as scientists, an integral part embedded in the team to connect the strategy with data. Share your insights and questions with your statisticians throughout. One of the mistakes people make is to only approach the statistician when they need sample size, tables, figures, formatted data or generally when there is something wrong. Instead, you should involve them throughout, from conception through interpretation. It is not just about sample size when you are designing a clinical trial at the start and it is not just about the tables and figures at the end of the study. It is about asking the right questions and getting the appropriate insights. Create the opportunity for your statisticians to work with the cross-functional team, hearing and contributing to the questions and insights, so that your statistician can use the right tools to help you.
"One of the mistakes people make is to only approach the statistician when they need sample size, tables, figures, formatted data or generally when there is something wrong. Instead, you should involve them throughout, from conception through interpretation."
How might a statistician approach study design differently than a CMO?
We bring more statistical perspective to the table. We assess if adaptive design or interim analysis is statistically sound and feasible to expedite the decision-making and save on cost. We challenge the team to prioritize the many objectives in the study, so we can generate the appropriate statistical testing strategy to avoid false-positive claims at the end. We believe in quality by design, so we actively mitigate the risk and improve the strength of our data package by building a missing data handling plan and finding ways to reduce missing data overall.
What are the biggest mistakes and misunderstandings that you see CMOs make around statistics and biometrics?
As I said earlier, silos are the biggest hurdle for us. Getting statistics and biometrics involved in the data operation is obvious (hopefully) but getting us involved in the strategy is more meaningful, ultimately leading to a better team and decision making.
For example, within data operations, we make sure that we collect the appropriate data with good quality, mapping it following relevant guidance and regulation, and make sure we analyze it appropriately. Most CMOs focus on that part probably because it’s easy to track per timeline. At the end of a study, a typical question to a statistician is “Are the tables, figures and listings final?” and when we say “Yes,” they move on. But it should not end there. It is really about insights. What does each output tell you and why? Do they address the question? Or do we need another way to look at our data? Only by having this type of conversation with your statisticians can they contribute and help the team generate better insights. If you want to get the most out of your statisticians and biometrics team, involve them throughout from generating the right questions to making decisions for next steps.
What are your thoughts on outsourcing versus insourcing statistical expertise in emerging biotech?
In my mind, whether you are big or small, it should never be 100 percent in-house or 100 percent outsourced. Specifically with a smaller organization, the data operations part – that middle part – needs to be outsourced because with limited resources, you should focus on strategy and vendor management.
As the organization grows, typically you bring more in-house statisticians as you organizationally have more studies in various phases and need different types of expertise to execute and design them. At that time, you may also be fortunate enough to have more resources to recruit, train, retain and motivate talent. As you move to later stages of development, you also need to build more quality controls to become inspection ready. Plus, having more in-house capability will give you much desired flexibility. For example, the in-house team can look at the data in specific ways in days if not hours, while the CRO model typically requires weeks or months of heads up to plan their resources and get their army ready. CROs are good and cheaper for batch work, but an in-house team is needed to fill in the gaps and oversee their work. That being said, you should probably never have a completely in-house model because every change in the organization would leave you with either too many or too few employees.
"When you do not have internal technical expertise, you do whatever the CRO tells you to do. Even the best CRO – because they do not really know your organization and do not have so much time to focus on you – may not have the right solution for you."
You were the first statistician hired by Keros. What was that experience like?
You should not be surprised by what you see as the first statistician in the organization. I started with data operation when I arrived because that is the first order of necessity for the organization. Only with that in a decent shape do statisticians have the credibility to meaningfully contribute to strategy. We had four different vendors providing biostatistics and programming services. As a small organization, that meant dividing a small pie into even smaller pieces. The CROs were not giving us much attention and even if they were, managing all the different vendors together takes resources and time. I consolidated by finding a vendor that could provide the entire biometrics service which made it both easier to manage and much cheaper. Specifically, I had one point of contact instead of four, a designated CRO team to support me and could build a relationship along the way.
Also, when you do not have internal oversights and know-how, you do whatever the CRO tells you to do. Even the best CRO – because they do not really know your organization and do not have so much time to focus on your projects – may not have the right solution for you. For example, after I joined, I spoke with people who had 20-30 years experience in the industry and no one knew the electronic data capture system that we were using. Having an EDC like that means that very few people know how to build or amend this infrastructure, and your timeline is at their mercy. We are transitioning to a much more popular EDC which makes it easier for us to find programmers to make changes quickly. It also makes it easier for the site, which, in turn, reduces the timeline and improves data quality.
Are there new trends in the biostatistics, biometrics and data operations space that CMOs should be thinking about?
It is always about improving efficiency and making better decisions. From the biostatistics world, this is not very new but adaptive design is a broad term that means that you can modify your study design based on what you learn both from the study itself and even external data. That can mean increasing sample size or treating a different subgroup of the patient population. You need to speak to a statistician to understand how to build that flexibility into your protocols. Master protocols are when you try to answer questions about multiple indications or compounds, rather than just one or two in a traditional clinical trial. It can increase operational efficiency but also increases trial complexity. Bayesian is a statistical methodology that is becoming more and more popular. Bayesian’s concept is similar to adaptive design in that you keep learning and updating and deciding the next step, as opposed to frequentist which is the most popular design in the past (and current) clinical trial world. At this stage, Bayesian is used mostly in early phase oncology studies, where you often have a small sample size to make a decision, like for dose escalation studies. People use Bayesian in later stage pediatric studies as well. They leverage the data for pediatric study decision-making because it is harder and ethically questionable to enroll pediatric patients. Other trials use real world evidence from outside of the study. For example, it may be unethical to have a control arm so you can use RWE as a historical or synthetic control arm.
On the data quality side, nowadays there are more and more data collected in clinical studies and traditionally they require extensive documentation and source document verification. Pre-COVID, people would go to the site and compare the document with the EDC. This is expensive, time-consuming and generally inefficient. Nowadays, there is a trend of moving towards digital technology, where data is entered directly and uploaded to the cloud for real-time access. This includes risk-based monitoring to reduce the SDV. On top of that is central statistical monitoring where you monitor for trends in data quality based on the data generated in real-time. The fanciest statistical methods are using artificial intelligence and machine learning in the central statistical monitoring.
"There are so many novel methods out there but some have only been done on paper while others have actually been used and speaking to a regulator may help you uncover the reason why and inform your design strategy."
What is your advice around best practices around clinical trial design and data collection?
For clinical trial design, talk to regulators often. Do your homework because there are lots of clinical trials already done. I often get surprised and inspired by the new designs that people were already using in different therapeutic areas. There are so many novel methods published, and some have been used successfully in the real world. Speaking to a regulator may help you uncover the reason why and inform your design strategy.