The Role of Generative AI in Making Better Immuno-Oncology Therapeutics
Alexandra Snyder, MD, tells us how she is overseeing a portfolio that combines generative AI, biological engineering and medicine to create new therapeutics for patients in IO. She is is EVP, R&D for Generate:Biomedicines, overseeing all clinical matters.
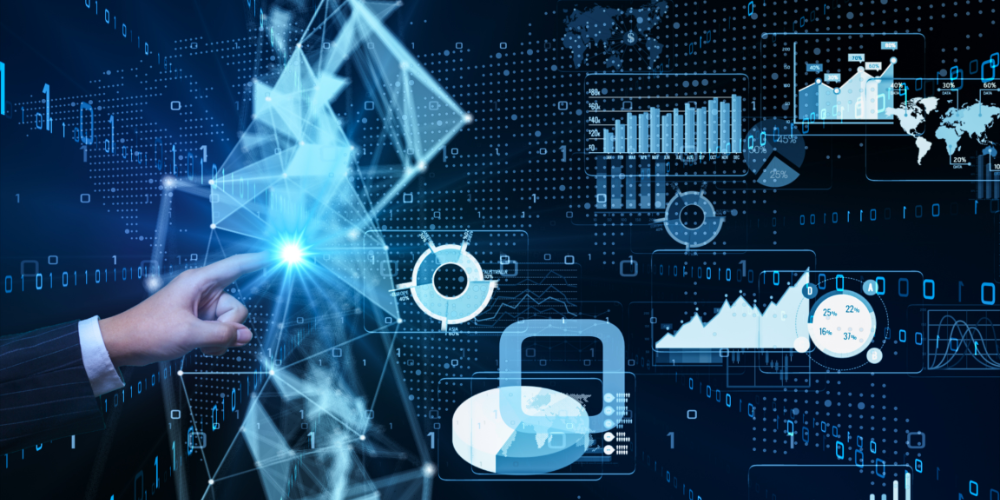
How are you combining AI and drug development to create new therapeutics?
Our company specializes in using AI and machine learning to make novel biologics. Through what we call a learning loop, we figure out a scientific or clinical problem that we want to address and then we ask the computer to propose solutions, which we then test in vitro. My role as Head of R&D is to lead the areas from the start of protein generation through clinical development. The three therapeutic areas we focus on are oncology, infectious diseases, and immunology.
"In this era when AI is very hot, I always go back to: What exactly is AI doing in this situation and what hypothesis does it support testing?"
What does that AI machine learning loop look like in practice?
If we wanted an antibody against a non-mutating portion of COVID, for example, the computer would propose solutions. We would then physically make the proteins in our wet lab. We test them at high-throughput against virus variants, as well as for the other attributes that a drug needs to have.
We put the data back in the computer, identify what it got right and wrong, and iterate upon that. It comes up with additional solutions until it reaches the target profile that we’re looking for.
Specifically for oncology, what is your strategy and focus?
Our approach is to decide what modality is needed based on the problem that we're trying to solve. In oncology, there are some situations where a monoclonal antibody is the right fit, but there are many in which you want something different: a T cell engager, or an ADC, or a cell therapy.
We can test whatever we do at very high-throughput, testing multiple parameters at once. That’s important for therapies like T cell engagers or cell therapies because each of these structures have various elements that you can adjust. For example, you can change affinity. You can change the relationship of the arms of a bispecific. You can change the signaling domain, in the case of CARs.
"If we can unlock de novo targeting – the ability to build a molecule from the computer that binds exactly where you want and does exactly what you want when it gets there – I think we'll be able to change drug development."
What does combining AI, engineering and bioscience solve in terms of what we can target?
The technology enables us to go after targets that we think – based on either preclinical or translational data – are good, but that the field hasn’t had the tools to pursue before.
For current methods for discovering antibodies or binding moieties within a larger structure, we're reliant on the immunogenicity of the target because we're injecting an organism to identify a binder. But in our case, we can either take existing binders that aren’t very good and make them better, or develop binders straight out of the computer.
What does combining AI, engineering and bioscience solve in terms of how we can target?
The computer is suggesting the solutions and we can measure the functions and behaviors of the proteins we make to ensure that those solutions are actually achieving the goals. For example, beautiful work has been done in the field of TCEs, ADCs, and CARs on what makes a good drug. We're learning about specificity and relative affinity of each arm; we have tremendous control over those.
Beyond that, in conventional drug development we typically have to do everything sequentially. Identify a binder, stick it together with a payload or other structure, test if it works in a dish, then in a mouse, then evaluate if it’s developable. With Generate:Biomedicines’ technology, we're optimizing on those things all at the same time. It shaves down the timeline considerably. We ultimately want to get something good to a patient quickly.
"AI can find patterns that humans can't detect. But to have that be useful, you have to give it patterns that are meaningful, at a scale that are meaningful."
How does the emphasis on machine learning, biological engineering and medicine change your role as EVP of R&D as compared to any other biotech’s EVP of R&D?
The primary impact of this emphasis is that data is at the center of everything we do. The Generate Platform integrates computational and functional data, and because we go in a loop several times, we have really diverse expertise around the table from the very beginning of the process all the way through to drug candidate. This means that someone like me, whose past experience is in translational and clinical, gets to have input from the very beginning about what we’re designing and defining our goal molecule.
How do you ensure that the use of AI is being appropriately applied?
In this era when AI is very hot, I always go back to: What exactly is AI doing in this situation and what hypothesis does it support testing? In our work, the hypothesis AIsupports is whether or not we can make better drugs faster. Can AI help us to make drugs against molecules that couldn’t be targeted before? Can we address targets in ways that are optimal for the ultimate clinical outcome?
AI can find patterns that humans can't detect. But to have that be useful, you have to give it patterns that are meaningful, at a scale that are meaningful. You must know what you're going to do with the pattern, especially because clinical data is very messy. We’re not in a “slam dunk” era of AI, but we’re in an era of change.
If we can truly harness the use of generative AI, what do you believe is possible?
If we can unlock de novo targeting – the ability to build a molecule from the computer that binds exactly where you want and does exactly what you want when it gets there – I think we'll be able to change drug development. In essence, our goal is to engineer biology. It is a lofty goal, but we've had incredible science done over the last few years, especially since the TCGA, and then the protein-based follow-ons.
There are a lot of targets and mechanisms to go after, we just need the ability to pursue them. We want to put molecules into clinical trials that have a higher probability of success so we can expose fewer patients and get to proof-of-concept quickly.
"We want to put molecules into clinical trials that have a higher probability of success so we can expose fewer patients and get to proof-of-concept quickly."
What do you see as the broader focus for the IO community right now?
Our focus as a field is shifting now from turning off/on or upregulating the immune system to actually trying to take control of the immune system and direct it. We all thought we would work through potential targets on T cells and the macrophages, and turn on or off the appropriate receptors to get better and better results. That strategy has not been as successful as the field hoped. In the meantime, other immunomodulatory mechanisms like cell therapies and immune cell engagers have started to blossom. The immune modulatory effects of ADCs, both alone or in combination with immunotherapy, have also become clear.
In the last few years, we've learned so much about these newer mechanisms that are helping us to design better drugs. I hope that we're at the edge of another burst forward in solid tumors that we haven't seen since the 2010s.
What are the specific modalities or pathways that you’re keeping an eye on?
First, in the immune modulatory space, I hope that we are on the edge of TCE starting to work in solid tumors in a more predictable and widespread way.
Second, I believe CARs in solid tumors are going to come of age as we work out what to modulate in the immune microenvironment and how the cell signaling may need to be different from liquid tumors.
Third, intracellular mechanisms beyond the small molecule era are on the horizon but not quite ripe. What are PROTACs and other molecular glues, or intracellular modifiers of signaling going to enable? Will they be able to get around the mechanisms of resistance that small molecules suffer from? And delivery goes hand-in-hand with an intracellular. How do we get into tumors, and once there, how do we get into cells?