How MIT Developed Invisible Remote Monitoring to Enhance Research
Dina Katabi, PhD, describes an entirely contactless experience for patients, with opportunities for earlier and better diagnosis. She is Thuan and Nicole Pham Professor of Electrical Engineering and Computer Science at MIT, and president/founder of Emerald Innovations.
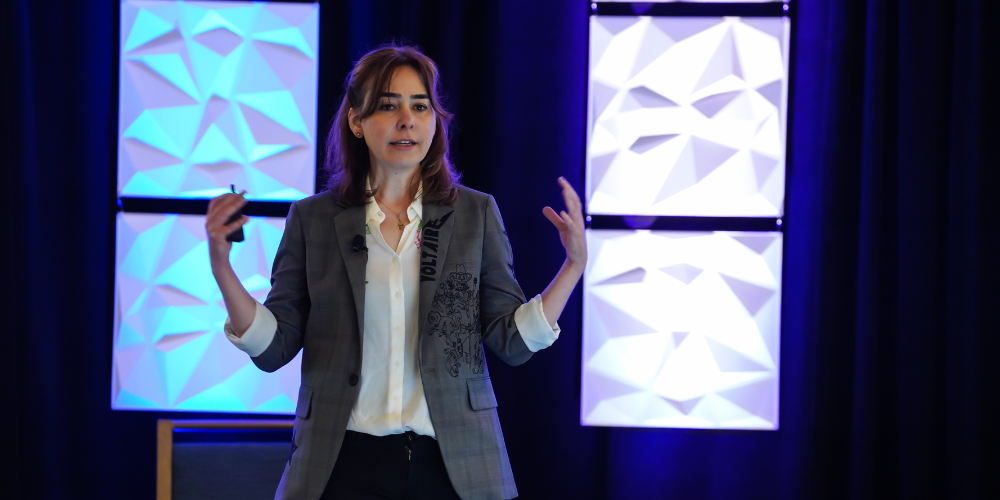
Can you describe how the invisibles work?
The “invisibles” are AI-powered sensors that sit in the background of the home like a Wi-Fi router, and analyze the radio waves that bounce off people’s bodies to measure their breathing, heartbeats, movements, sleep, and behavioral symptoms. Invisibles measure these health metrics while people simply go about their lives in their homes, without requiring them to wear any devices or interact with the sensors.
What disease areas have you applied this technology in?
Our sensors are used by pharmaceutical companies and health organizations to track nocturnal scratching in atopic dermatitis, movements and disease progression in Parkinson’s, behavioral symptoms in dementia and Alzheimer’s, etc. The sensors are also used in a variety of autoimmune and immune diseases such as Crohn’s and Lupus, and rare diseases such as Rett Syndrome and FSHD.
What do you see as the practical application of the invisibles work you’re leading?
We see them as having a major benefit both for pharma/ biotech companies and in the broader healthcare space. Pharma and biotech companies are increasingly interested in decentralized clinical trials, i.e., moving clinical trials to the participants in their home, rather than the current model of bringing participants to clinical sites. Such distributed trials could reduce the overall cost of clinical studies, and make them more accessible to participants for whom access to clinical sites might be challenging due to socio-economic conditions or simply, geography.
But in that context, there are two things to be aware of. First, there is the risk of overloading the participants (for example, with ensuring that they wear their wearables, charge them regularly, upload the data etc.). Second, there is the compliance you need from the participant when you are trying to collect data using Patient Reported Outcomes (PROs) via digital health technologies.
“Invisibles remove both the burden and compliance concerns - patients just have to live their lives normally and the data is collected without requiring them to do anything out of the ordinary."
And how does your work mitigate those two challenges?
“Invisibles” remove both the burden and compliance concerns - patients just have to live their lives normally and the data is collected without requiring them to do anything out of the ordinary. And because the invisibles collect data all the time, rather than just once every few months when the patients go to the clinic, they can obtain many more measurements in a shorter period of time, potentially reducing the variance and ultimately the time to obtain statistically significant results from a clinical trial.
What impact would this technology have on how we deliver care?
It has the potential to make dramatic changes to our current model of delivering healthcare, especially for chronic conditions and higher risk patients. Today, the healthcare system relies on periodic check-ups of patients by their providers, and for patients to contact their providers if they feel that their condition is getting worse. Most patients do not seek medical attention until it is too late, leading to ER visits and hospitalizations, which are both expensive, and lead to overall poorer health outcomes.
It is also not efficient, since many individuals do not have any major need for additional care revealed in their regular check-ups. In contrast, the ability to collect data continuously and track changes in patients’ health as they just go about their lives, and trigger alerts for either patients or providers when patient health deteriorates or their status changes, can enable providers to utilize their efforts to intervene in a timely manner for the patients who need it most when they need it.
What example can you give of new patterns or findings being discovered?
We have demonstrated an objective and sensitive endpoint for atopic dermatitis and itch. Today, itch assessment relies on asking patients to rank the severity of their itch on a scale from 0 to 10, where 10 is the worst. This approach is subjective, insensitive, and hard to use in a pediatric population. We show that the Invisibles can detect when someone scratches using the radio signals that bounce off the person’s body. This allows for assessing itch using nocturnal scratching in an accurate and passive manner. Further, since the Invisibles can measure sleep and sleep stages from the radio signals in the environment, we can also assess the impact of scratching on the person’s sleep. We can perform this assessment in the person’s own home every night without any wearable sensors, cameras, or diaries.
We’ve also shown that we have the ability to have both a diagnostic biomarker of Parkinson’s disease that has the potential to detect Parkinson’s disease even before the motor symptoms emerge. It’s also a progressive biomarker, meaning that it has high sensitivity and can capture the progression of the disease over a shorter period than trying to capture the statistical significance with MDS-UPDRS, which is the gold standard.
"It has the potential to make dramatic changes to our current model of delivering healthcare, especially for chronic conditions and higher risk patients."
Artificial intelligence has been applied to images and texts, but you’re applying it in a more novel way to radio waves. Can you tell us about the AI underpinning this monitoring?
Much of deep learning and neural networks have been applied to images, audio signals and text. Here, the innovation comes from being able to analyze this new modality – radio signal – with something as powerful as a neural network to extract information that is hidden in the signal and is not easy to extract using other methods.
This is quite powerful, because when you use neural networks on images, for example, you are trying to replicate an expert, such as a cardiologist or pathologist. But with radio signals, our invisibles can extract new information from their continuous monitoring that has so far not been available to human experts. In a recent collaboration, we show that being able to extract this new information allows us to detect Parkinson’s disease from someone’s sleep and nocturnal breathing. This result is exciting as it can potentially enable the detection of Parkinson’s disease at a very early stage before the appearance of motor symptoms.
What do you view as the progression of pharma utilizing data science more into its trial decision-making?
Pharma is ultimately very science-driven, and you see that different pharma companies are already taking steps to utilize data science in various aspects of drug discovery and development. Of course, data science and AI are newer technologies in the pharma world, and different companies are at different stages of adopting and integrating these new ideas. But, everybody recognizes that this is a necessity.
Today, many pharma companies are using AI in the preclinical phase, for example to design molecules and identify targets. Also, some pharma companies are using AI in screening patients and sites.
Where in the trial process do you see the value in utilizing technology like this?
One of the most valuable opportunities comes from combining digital sensing and AI to sub-type participants in clinical trials according to their physiological signatures, and characterizing how their physiological signals change in response to a drug of interest. Such subtyping in early phase 2 clinical trials could help in avoiding later stage failures, which are arguably the biggest challenge today in drug development. It can also be very powerful in expanding the drug to multiple indications. Many modern drugs, particularly in immunology, are not specific to a particular disease and interact with complex pathways. Collecting detailed physiological data about the participants across multiple clinical trials related to one drug or dependent pathways allows for a holistic view of the drug and its potential in a variety of indications.
How does invisible monitoring reduce late-stage clinical failure?
It enables us to understand the disease and sub-type the patients based on actual physiological signals, and how they change in response to a particular drug. We’ve shown how you can use physiological signals to separate and cluster different individuals in your clinical trials without even knowing or telling the machine how to structure them or subtype them. Machine learning discovered that there are underlying differences in the physiological signals. And in some trials, some of them could be your responders, and some non-responders. You can then say, “Okay, why did these clusters of patients respond?” There are many things that we can do that directly target how the drug impacts humans, as a result reducing the risk in the later stage of the pipeline.
For more information on DPHARM: Disruptive Innovations to Modernize Clinical Research, visit DPHARMconference.com.