Genentech’s Head of Digital Health on New Devices in Remote Monitoring
Thomas Switzer describes the strategy for overcoming company hesitancies and concerns when it comes to adopting innovative technologies, as the Head of Digital Health for Genentech Research and Early Development's Early Clinical Development Informatics.
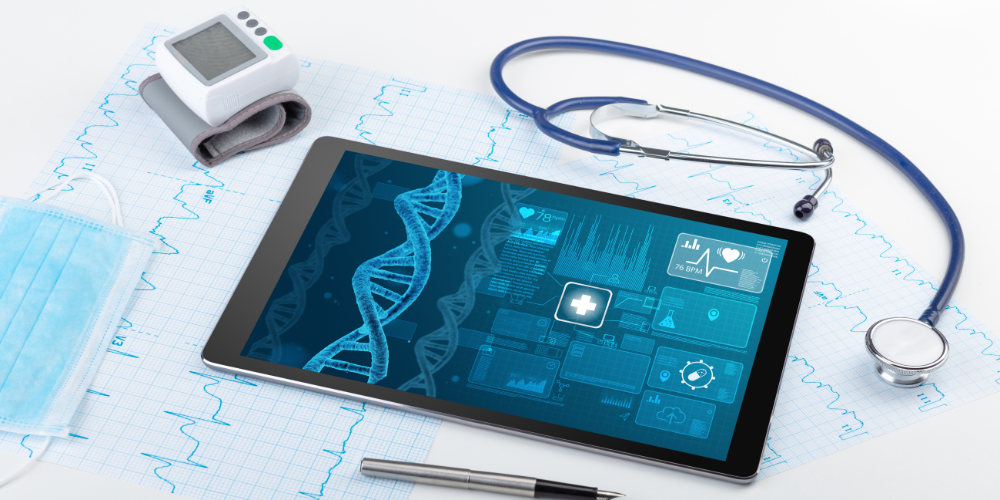
What has been your past experience in collecting and assessing data remotely for clinical research?
A lot of it has been in piloting to see if we can collect signals that look to be clinical-grade. More recently, we’ve been including more as exploratory endpoints, with the intent to actually position as secondary endpoints or make label claims off of the outputs of the sensors.
The other element has actually been replacing some of in-clinic assessments without home assessments, largely for clinical trial continuity in the age of COVID, or as an attempt to make trials more patient-centric, and more convenient for patients to participate.
What is the process to shift assessments from in-clinic to decentralized, while maintaining data rigor?
One key step is ensuring that the tool you use matches your clinical standards. You have to show comparability between the at-home measures versus the in-clinic measures, so that the data is interoperable. If you haven’t done that, the data is likely not usable. That has to be done through validation, to make sure that things are compatible.
That is some of the work that we’ve been doing. For example, we have looked at in-clinic cardiology versus at-home using a patch, to see if there is compatibility, operability and data quality. You want to see that there is not a lot of artifact – out-of-range physiologic variables – because sometimes these tools are syncing data much more frequently and you may see artifact.
"If you want to actually make meaning of something and determine that it’s not just a shiny object, you have to go through a stepwise validation."
What is the change in data analysis during the shift from collecting data at concrete intervals to more continuous?
In some cases, it’s really a data effort. It’s how you summarize that data and reflect back. You can sample heart rate at 60 hertz – 60 times a second – but your heart rate doesn’t change that fast. So in essence, you might have to summarize that down to five minute rolling epochs or 15-minute epochs, or one hour epochs, etc. When you come in-clinic, you get a single measure of temperature, so you have to decide how you want to condense and average that value now that you can capture it more frequently.
Some of it is also the design element. How frequently do you need to sample? Do you need to collect a patient’s heart rate on a minute-by-minute basis, or once an hour or once a day? You have to pinpoint the optimal frequency so that you aren’t getting a lot of noise or collecting a lot of unnecessary data.
For new devices, how do you begin working it into clinical practice?
That’s why we do pilot studies or as exploratory endpoints. If you want to actually make meaning of something and determine that it’s not just a shiny object, you have to go through a stepwise validation. That’s why Phase Ib, Ic or IIa space is a great place to start. It is a small subset; you can actually see how it works with your intended patient population. You have to do that pre-work there just to make sure it looks like we will get a signal.
We decided on endpoints – like safety endpoints or efficacy endpoints. We tested and built it up, with the idea that this would mature and follow a molecule through development. But we’re doing some of that early work outside as part of either validation studies or natural history studies, where we get a better understanding of how the instrument operates in the wild.
Has the attitude changed from being stuck in pilots to beginning to actually implement?
There’s a willingness to go beyond pilots, but there’s an internal dynamic that you need to overcome for that. That’s actually largely what I do. It’s analogous to “Not In My Backyard” – “Not In My Study.” People are interested until they actually have to put it in their study and take accountability for the outcomes, because there’s a lot of uncertainty about what you’re going to get out of this. There’s still cultural headwinds, but I’m finding far fewer of them now that we’ve actually socialized and used it a lot more.
There’s other information coming out from other forums, such as other companies that are using this. There may be a little bit of FOMO. Key is not to over-promise what a remote device is going to do. It’s useful in the context of answering scientific questions, as long as you formulate your questions really well, and you walk people through the whole process.
What does the assessment and deployment of digital remote monitoring look like?
The idea is when you’re designing a study, you can look at what the most optimal data collection and study design strategies are to get the needed data, and if it includes digital. In some cases, it won’t include digital. But if you are using digital, what is the intended endpoint: primary, secondary or exploratory? Are you trying to understand disease biology?
Studies are incredibly complicated. You have 15 stakeholders who all want something out of the study, and we come in as the 16th. Therefore, it’s always interesting to see the tradeoffs in study design – what you would like versus what you need to have – and how digital and mobile look in that balance.
Are you applying remote monitoring mainly to disease characterization, or do you support drug efficacy monitoring and adverse event monitoring as well?
It’s all of the above, actually. Roughly half of our portfolio is oncology-specific, and the other part of it is non-onc.
Oncology isn’t really interested in digital for efficacy endpoints, because you’re looking at progression or survival as endpoints, and these are well established. However, there is a lot of activity and utility in safety monitoring. For example, looking at things like cytokine release syndrome. You’re looking at changes in vital kinetics. Those are well-suited for wearable technologies, but again, you have to validate them for their accuracy. If a patient takes a patch and wears it at home, you have to show that you’re getting that accuracy there.
In the non-oncology space, because you’re dealing a lot with waxing-and-waning diseases or flare-remission diseases, this is where we start to look a little bit more at efficacy and prediction. We ask, “Can we see these changes to behavior patterns, improvements in functional abilities, using accelerometry? Does a person sleep better because their disease is better controlled, because they have better symptom control? And how do you document that?”
Are you able to incorporate more quality-of-life data from remote monitoring into studies?
That’s the idea: you get some objective data from a wearable sensor, looking at activity bouts or something like moderate- to-vigorous physical activity. You can then contextualize that with some PRO elements, like “I did move better, because I felt better,” etc, and objectively it’s reflected in, for example, total steps.
Fundamentally, these are tools to answer scientific questions. You have to understand if the question is safety-related, if it is efficacy-related, etc. The clinical scientist ultimately owns the study, so I help create these tools or work with them in support of the scientific questions. As long as we have a really good scientific rationale, then we can look at the technology.
"Studies are incredibly complicated. Therefore, it’s always interesting to see the tradeoffs in study design – what you would like versus what you need to have – and how digital and mobile look in that balance."
How are you using this for remote safety monitoring?
Sometimes for safety monitoring, we hospitalize people for three days for CRS monitoring. But with remote monitoring, perhaps you can get them out of the hospital. Maybe they’re in a local hotel, or something nearby where they’re being monitored, but they’re not in an intense clinical environment. They’re somewhere more comfortable while still dealing with a high-fidelity signal and still having the confidence that they’re being looked after.
Can you describe the STARMAP study and what it aimed to validate?
We wanted to get more insight into home spirometry versus in-clinic spirometry, in idiopathic pulmonary fibrosis (IPF). For IPF, one of the endpoints is a 10% decrease at the end of 52 weeks, and that is predictive of mortality within a certain set of time. The question was, “Could you use home spirometry to detect an earlier drop in Forced Expiratory Capacity (FEC)? And what does the data look like doing daily FEC single blows?”
We wanted to know if we could get more granular by using these data sources, and in the content of a natural history study, observe patients over time and get a sense of patient acceptance. And then, if we have another IPF study, we could include these tools with a level of confidence that they could give us the data we need.
What was the result of that study?
In that study, we saw a lot of noise. We felt maybe the ideal optimal sampling frequency wasn’t a single blow daily, but three blows once a week. We added in a wearable for steps and coughs, and we gained some subjective data about coughing intensity, frequency, etc. But overall, we decided that we didn’t have confidence in in-home spirometry data that’s clinically relevant enough to use as primary endpoints. We felt it was too noisy. But it was good to get that data, because we’ve never actually really tested it.
For more information on DPHARM: Disruptive Innovations to Modernize Clinical Research, visit DPHARMconference.com.