Forming a National Data Collaborative to Better Treat COVID Patients
Dr Melissa Haendel talks about her role in the National COVID Cohort Collaborative, the largest publicly available limited dataset in US history. Dr Haendel is the Chief Research Informatics Officer at the University of Colorado Anschutz Medical Campus.
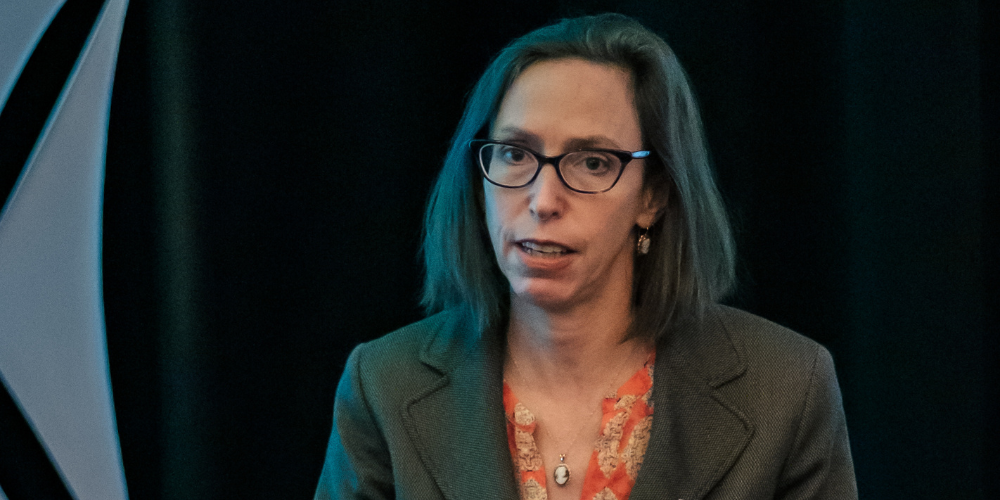
Part of your recent work was taking on the monumental project of forming the National COVID Cohort Collaborative. What's your background and interest in informatics?
I am the contact principal investigator for a coordinating center called the Center for Data to Health, which is the coordinating center for informatics across the 60 Clinical and Translational Science Award sites. The goal of that coordinating center is to try to advance data sharing and interoperability and collaboration. It’s no small task. We had been marching along on our various paths to trying to achieve some of those goals. But when the pandemic hit, we asked ourselves what we could do that would really help the nation address the pandemic that is different and complementary to what already exists. We didn’t want to duplicate effort; we were trying to make the most of all of our person power across the nation as best we could.
There are a number of distributed research networks that use different common data models such as PCORnet and OHDSI. They each have helped institutions put their data into a data model structure locally, behind their clinical firewalls. In a distributed fashion, you can ask questions across them all. That way, the data stays behind the firewall, but you can still ask questions across a large group of sites. Some of these groups have been very successful at doing that, so we didn’t want to duplicate that. The issue though is in that scenario, you know what questions you want to ask and that you can answer. So it’s much less able to do discovery-oriented questions. If I want to ask about how many patients with condition “Y” are on drug “X”, I can do that. But if I want to find positive and negative correlations between all the drugs and all the conditions, it’s much more challenging to do in a distributed fashion.
Can you describe the process of pulling those sites into a common infrastructure?
We partnered with the four primary research networks. In that way, we aligned the different common data models and allowed push of data from any site that has their data in any of those models. It democratized the models, so that any institution could participate if they had their data in one of those models. One of the things that we’ve noted is that even within a given model, different sites are populating those models differently. We found a lot of different data quality issues that were revealed by aggregating the data, that you didn’t really know was really such a problem in the distributed context because you never see the data as a whole like this. In that way, everybody’s data has been getting better.
The other piece of this in terms of regulatory oversight, we partnered strongly with NIH to figure out how to get the regulatory oversight to be able to have the institutions transfer their data, as well as to provide broad access with institutional data use agreements to anyone who has completed human subjects training and security training, and can be vouched for by their institution. That goal was to support anybody who might be an expert in machine learning, but knows nothing about clinical data, or clinical experts working together. It takes a village to analyze these data. You need clinical expertise, statistics, machine learning, data modeling, clinical data informatics, etc. We really worked hard on the regulatory components to make sure that we could provide broad access.
The third part was that NIH had an already instantiated FedRAMP-certified secure data enclave where we could push the data in the early part of the pandemic. It takes almost two years to get FedRAMP certification for the security review. If we hadn’t had the right analytical platform already in place with FedRAMP certification, we would never have been able to create the enclave. We’re up to 82 institutions now that are pushing data. It’s broader and bigger than the CTSA community in which it started, and includes IDeA-CTR organizations and other local and regional health care organizations such as OCHIN. This is wonderful as it ensures that the cohort is demographically diverse and representative of our nation.
"Doing better research studies is one of the key ways that data can be used effectively."
You describe your vision as weaving together healthcare systems, basic science research and patient-generated data. How does informatics bring clinical research closer to clinical care?
A lot of my work is in the rare disease space where we really have a requirement to engage patients in their own care and in the research. They’re very enthusiastic participants. That’s one of the one most wonderful things about the rare disease community. A big component is the way that rare disease patients are really engaged in the research both on the clinical side and the basic research side.
In the context of the N3C, we are working with a patient group that has just been a really great emerging partnership. They have created us a survey and advocacy for “long COVID” patients. And we’re going to be working with them to pull in patient-reported information alongside the EHR data, as well as other kinds of data types, such as wearables, that would come directly from the patients. We’re including them in our analyses of the data and thinking about what their needs are in terms of having information come back to them.
One of the hopes of the N3C is to say, “We pulled our data and we found these results or analyses,” or “We’ve made these tools or predictive algorithms. Let’s deliver that back to the clinical site so they can use it.” If it means that “X” drug exacerbates COVID outcomes, stop using that drug.
The same thing is true for the patients; what the patients get out of it. We want to make sure as they’re reporting their symptoms and interacting with systems that help support them, that they get information back that helps them think about how they fit into the context of the greater data that’s being collected as well.
For example, when you fill out a survey, and it says, “75% of users answered the same way as you.” There could be something like that, which helps the patients understand how common or how unusual their progression is versus the other patients in the system. Especially if they have an unusual presentation, to understand what to do about it, and how to make sure that’s logged, that people get back to you and that there’s a strategy for understanding that.
We believe these types of interactions can lead to a much richer dataset for us to integrate with the clinical data, to provide a much more complete picture of the patient that we can analyze to help better classify patients into categories. That can help us better decide what their care and treatment should be.
"You cannot find results from these data as one person. You have to have very diverse expertise working together in order to ask the hard questions, find answers and make sure that they’re robust."
Could it be used in a post-COVID context?
That’s something we’ve been working on trying to figure that out. We do believe that the structure of doing this has been revolutionary for informatics across the US. It’s the largest publicly available limited dataset in US history. It’s a phenomenal degree of partnership and sharing across different communities and institutions. The infrastructure for the harmonization has been built in rapid fashion with the partnership of those common data model research communities. That could readily scale to doing all EHR data and all disease areas, but the regulatory requirements for that would be different. This data was transferred with the sole exclusive purpose of being used for COVID. As long as you’re studying COVID in some way, the access is pretty straightforward.
We do believe that this could scale for many different things. There are groups applying for grants to see if they can use the infrastructure that has been built, but for different disease areas and with different regulatory agreements. Simultaneously, conversations are ongoing across NIH and across the institutions about how wanting to grow this capacity for the ability to be used by the community for all diseases.
That’s where we would like to head but there are a few regulatory barriers. In addition to that, will people feel the same about data-sharing when the pandemic is over? We hope that this has changed the culture, and that we demonstrated security and safety of sharing data in this way, so that people will feel more confident in doing that more broadly for future research.
What guidelines would you like to see in order to make full use of the data we are generating, including from newer sources of data?
Two comments. One has to do with how we design trials and studies. For COVID research, or any new disease, we often start without a good definition of what constitutes somebody with that disease. “Who has long COVID?” is our question of the day, for example. This is where that patient-matching classification comes in. There isn’t just one long COVID patient, and they’re not all necessarily suitable for the same research study.
Research studies are expensive and time-consuming; we want to make the most use of those resources. We need to be using observational data from the EHR, but also from the patients, whether it’s wearables or patient surveys, to better inform how to classify patients into different subgroups that can then be assigned to the right research study. Doing better research studies is one of the key ways that data can be used effectively.
The other thing is a long-term strategy of team science around translational analytics or collaborative analytics. You cannot find results from these data as one person. You have to have very diverse expertise working together in order to ask the hard questions, find answers and make sure that they’re robust, clinically and in terms of data science. We’ve been working on coming up with “domain teams” to help support people, projects and teams to make sure that they have the expertise they need to do good science and to do efficient science. Make science go faster, better.
To learn more about Clinical Research as a Care Option, visit CRAACOevent.com.