Eisai’s SVP of Biostatistics on the Bayesian Trial for Early Alzheimer’s Disease
Eisai’s Bayesian Phase IIb study dose-ranging lecanemab led to the design of the Phase III Clarity AD trial to verify the drug’s clinical efficacy and safety in early Alzheimer’s Disease. Shobha Dhadda, SVP of Biostatistics and clinical development operations for Neurology at Eisai, describes the unique hurdles that accompany Bayesian designs.
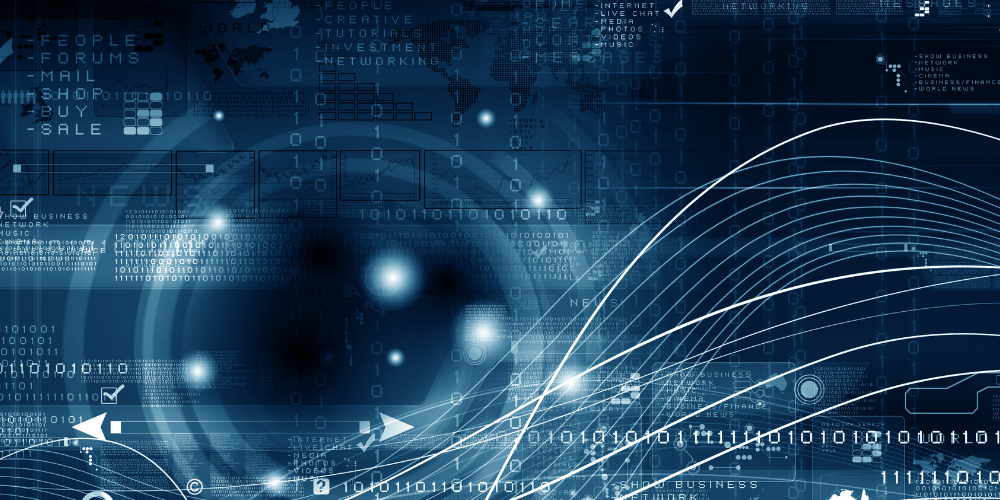
How does your role change when you are part of an adaptive design or some other form of an innovative trial design?
In a traditional design, you have fixed assumptions on treatment effect, variability in the study, dropout rate etc. Using these assumptions, you can calculate the sample size. Then you perform simulations under different scenarios to make sure the sample size you're proposing for a standard design continues to have robust power to determine if the drug actually works. Once you fix the design parameters (sample size, treatment effect, duration of study), study is conducted with these fixed trial design parameters.
But when you're working on an adaptive design, you have to think differently, especially during the design phase of the study. You don’t have any fixed parameters. And usually when you’re running an adaptive or innovative design, you’re doing so because you either want to gain efficiency or because there are so many unknowns that you cannot control in a fixed trial design. In that case, you want to have the opportunity to be able to adapt based on the data collected. This is generally implemented through machine learning computer algorithms and computer systems for treatment allocation so as to not impact the double-blind nature of the study. This has to be carefully implemented with adequate system controls and firewalls.
"When you’re running an adaptive or innovative design, you’re doing so because you either want to gain efficiency or because there are so many unknowns that you cannot control in a fixed trial design."
What does that mean for the simulation and pre-planning?
It means you must first perform thousands of simulations to just design the study. These simulations must cover all possible scenarios to ensure you understand the probability of success and failure comprehensively. Then you must put in operational aspects to make sure the conduct of the study goes smoothly, and integrity of the study is not compromised. It's very intense.
The lead statistician for the study must look not only at the initial assumptions but also the universe of possibilities, and make sure that you're still going to be able to predict the probability of success at the end of the study. Clinical team needs to fully understand the impacts to power and type I error.
How does a platform approach impact sample size?
The advantage of platform studies is that you're setting up an infrastructure for a similar disease area, such as early Alzheimer's disease or COVID-19. When it’s the same disease area, you’re looking at a similar patient population. You are able to share the infrastructure, and share the placebo. That itself is reducing your needed sample size, depending on how many drugs you are testing.
On top of that, because you are adapting the study as you go along based on the data that is accumulating, you could hit your success criteria or failure criteria earlier. That allows you to either cut your losses early or move to the next stage.
And in terms of lost-to-follow-up, it should be irrespective of arm. The only reason you would see more or less dropouts on a platform, would be because of lack of efficacy or one drug has more adverse events as compared to another, or an unexpected adverse event that is not common in the disease area. For example, infusion reactions could cause one arm to have more dropouts than the other.
What were the unknowns you were concerned with in the Phase II lecanemab study?
For the clinical trials in the past that had failed, the unknowns were not having the right hypothesis, or the right dose, the correct study duration, or magnitude of treatment effect, or even the right patient selection criteria. Clinical trials were moving towards an earlier stage of the disease. We had five dose regimens of Lecanemab, with the goal of identifying the most effective dose that could be taken to phase 3. Because this is a slow-moving chronic disease without completed Phase III studies, we didn’t know if the endpoint could be or should be at 12 months, or 18, or 24.
From a practical point-of-view, If we had run a traditional Phase II study, we would have needed at least four times the sample size, which would have been a lengthy and costly study and would have exposed many more patients to ineffective doses.
Because of all these different unknowns, we decided to use a Bayesian adaptive design and make sure that we could run a proof-of-concept study, while mitigating all the risks. The Phase III study replicated the patient eligibility criteria, used global sites and used the dose selected and treatment effect assumptions based on the Phase II study; the results for the Phase III study actually replicated what we saw in the Phase II study, both in efficacy and safety.
"We learned a lot from the Phase II study design and many of these things, even in terms of operational biases, we implemented in the Phase III study."
What are the challenges in terms of bias in an adaptive or multi-arm trial?
In any clinical trial, there are statistical biases to account for, but in adaptive design, there are additional operational biases. Those could be perceptual, and some could be actual. As an example: functional unblinding. If a PI in the study knew what the cognitive assessment was, could they bias the results? Or looking at who made the decisions of the interim analysis? Was it being handled by an independent monitoring committee? What was the sponsor's role in it in this whole setup? What was the data flow during these interim analyses? You must look at if there was anything that could have introduced additional bias during the conduct of the study.
You have to have many analysis plans and charters in place. Instead of just having a statistical analysis plan, you need to have a simulation plan. You need to have a data monitoring committee charter. You need to have a data integrity plan that defines the data flow. These are necessary to mitigate the risk of operational bias.
Another important challenge is how to implement response-adaptive randomization in a clinical trial, where a pre-built computer algorithm assigns more subjects to more effective doses. This computer algorithm needs to be built before the study is started, before a single patient is screened in the study. It takes several months to build it. Once built, this computer algorithm has to be implemented such that it is completely handled within the computer system that randomly assigns treatments to patients in a clinical trial so that the whole process is managed in a blinded manner with no involvement by the sponsor, CRO or site.
"Everyone needs to see the success stories. That's the only way they can understand that it doesn't have to be one-size-fits-all when it comes to study design."
What are some of the unexpected challenges that arise from using adaptive designs?
It can be much more difficult to explain to internal and external stakeholders, and to regulators. And even when you have the results, it can be difficult for clinicians to understand how they can interpret those results.
In the past, I have done sequential designs. They are also innovative, and they have similar operational biases to adaptive designs. The difference is that regulators understand this design more, because you can derive things mathematically. You can say, “This is my level of testing at interim one. This is my level of testing for interim two. And then finally, this is how I'm going to make sure I have a p value less than 0.05, which is when Type 1 error is controlled. In a Bayesian setting, all of that is done using simulations, and thus is more complicated.
What did you do to combat that difficulty?
We have internal training sessions within Eisai, including key stakeholders or advisory boards. Externally, we had the design paper published and we shared with regulators multiple simulations under multiple scenarios to show them how robust this design is. But it's still very difficult. But the regulators are coming around; we have draft guidances in place. It's very common now in oncology, so hopefully other disease areas will catch up.
And because we were the first ones to run this Bayesian design in a disease area as difficult as Alzheimer's disease, there are still people who have difficulty in understanding it. We had key opinion leader meetings; we had scientific meetings. We put this movie together to show how our design works. It answered questions like “When does it reach a futility boundary? When does it reach a success boundary?”
"There are many approaches and depending on the need for your particular study, there are solutions. And it’s key to evaluate all possible designs before making a decision on what the right design is for your study."
How has your experience in Bayesian designs impacted your approach to traditional designs?
When you're doing Bayesian designs, and you understand what must be done to ensure project success, such as multiple simulations, you start to apply those concepts to traditional designs as well. It doesn’t matter what the assumption is, you want to make sure the study design will ensure you’re able to see statistically significant results.
I can go back to the lecanemab example. We learned a lot from the Phase II study design and many of these things, even in terms of operational biases, we implemented in the Phase III study. We had to make sure that we could address any question regarding functional bias or operational bias so that when it was time to interpret the results from the study, we were able to address every single question related to study conduct.
Do you have a key learning about running the operations of an adaptive trial from the Phase II lecanemab study?
The important key learning for me was to conduct a Phase II study before moving to a large Phase III study, especially in these difficult-to-treat disease areas. And in that Phase II study, make sure you understand what the unknowns are, so that you’re able to design a study to address those unknowns in a robust manner.
For example, if your key issue is that you’re confident about efficacy but not safety, a seamless Phase II to Phase III design may be one good approach. But above all, keep an open mind to design alternatives.
How can we move towards greater uptake of innovative and adaptive study designs?
It comes back to education, but not just for the statisticians and the clinicians. It's the therapeutic area. It's the external world. Everyone needs to see the success stories. That's the only way they can understand that it doesn't have to be one-size-fits-all when it comes to study design.
There are many approaches and depending on the need for your particular study, there are solutions. And it’s key to evaluate all possible designs before making a decision on what the right design is for your study.
For more information on DPHARM: Disruptive Innovations to Modernize Clinical Research, visit DPHARMconference.com.