Cleveland Clinic’s First Chief Research Information Officer on Streamlining Patient Recruitment Efforts
Lara Jehi, MD, discusses synthesizing fit-for-purpose data to streamline patient recruitment efforts in multi-center trials. Dr Jehi is the Cleveland Clinic’s first Chief Research Information Officer, where she oversees the clinic’s robust research informatics environment to advance biomedical research throughout the system.
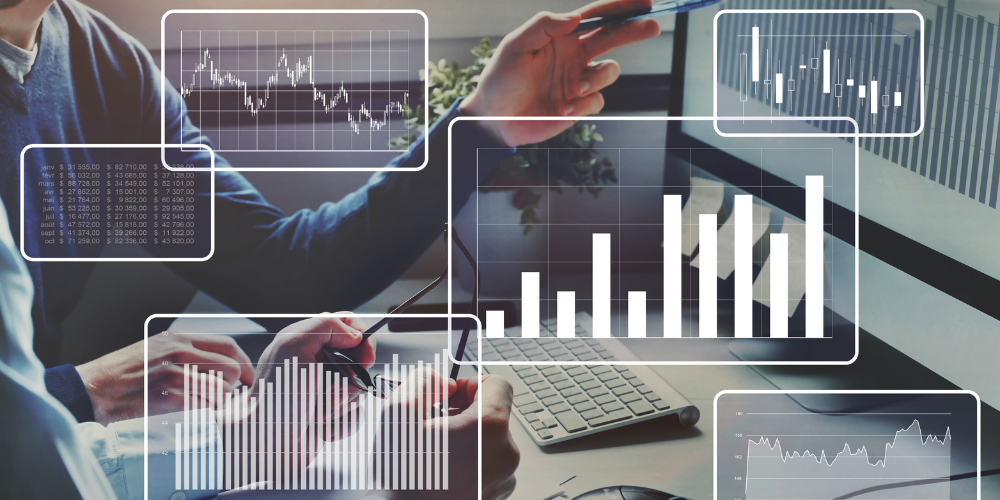
With more and more data available from wearables, EHRs, etc, how do you begin to synthesize in order to provide patients with the best access to care?
One can get overwhelmed very easily. My strategy is the same as in dealing with any big problem: it is best to stay focused on the goal. Define the goal first, define the “why” first, and then worry about the “how” and “when” and get bogged down in the details. As I get approached with all types of studies that want to use any one of these different forms of data, the first question I ask is, “What’s the research about?” What’s the question about? Is it something that’s clinically meaningful? Is it really going to make our patients’ lives better?
Depending on the answer to that, then I move onto, “Is this the best way to do it?” Big data looks attractive on paper, but sometimes there is a better way of answering the question, using more traditional approaches. And then, what is the safest way of doing this? We have to keep patients’ privacy and confidentiality – the regulatory pieces – in mind.
With all of these big efforts, there need to be teams that are organized around addressing each one of those different pieces. It takes a team of stakeholders, subject matter experts, to be vetting each one of those questions.
One of your roles is in the utilization of EHR data to streamline efforts of patient recruitment for multi-center trials. What are the challenges and opportunities of that?
Pre-COVID, The challenges were how to translate definitions of data pieces that you’re interested in, across organizations. The way we were doing it pre-COVID is, as part of the National Trial Innovation Network, investigators who wanted to do multi-center studies worked with a TIN to develop a standardized EHR query that would help identify patients who could fulfill inclusion/exclusion criteria for their studies. Then we would get those, and I had a small team of analysts within our enterprise analytical structure in Cleveland Clinic. They would help perform these queries and identify, at least on a big picture level, which patients would be theoretically good to approach for a given study.
The challenges that we had with that are that no matter how hard you try, it’s hard to translate inclusion/exclusion of a given protocol into an electronic health query. Oftentimes, we found that we were overestimating. With these EHR feasibility queries that you often have an overestimation of potentially how feasible your study is.
One way we dealt with it was that we went about it the other way around. Instead of generating these queries in bulk, our teams who were focused on doing research, we used that programming to alert physicians on the spot, through a best practice alert that would come up in Epic, that this patient would qualify for Study X. Then it was up to the physician then, on the spot, to decide if they wanted to approach the patient or not.
Post-COVID, of course, all the walls fell down. Everyone wants to share data. We have been able to put together a team to build a clinical registry for all of our patients tested for COVID-19 in the Cleveland Clinic. Pulling pretty much 98% of the data automated from Epic, through queries that our team did, we have more than 23,000 patients in the registry, with more than 300 variables on each patient.
The message here is that what COVID taught me, is that the best way of using the electronic health record for clinical research and for clinical trials, is to have a combination of an efficient, automated way of getting the data out, but also an intellectual piece of speciality- driven, human curation of specific data elements, to make sure that you get as clean of a dataset as you can. You do that human piece at the beginning, to validate and verify each one of your data elements; after that it can all be automated. But without taking the time to clean it up front, I think it’ll be garbage in, garbage out.
"Post-COVID, of course, all the walls fell down. Everyone wants to share data."
What could data informatics and research look like on the primary care provider side or for the patient?
It should look like clinical care. The ultimate goal is to have this research facilitating clinical care. On the spot, on the fly, as the patient is getting their care. It shouldn’t live in its own dungeon and clinical care be going on separately. The two should be parallel, aligned, going hand-in-hand.
One example is these best practice alerts. A patient and a physician are both made aware of a research opportunity in the context of a routine clinical visit. Another mechanism that we use to make research integrated into clinical care is the individualized prediction model that we’ve developed.
To give an example, we just built a predictive model that can identify a patient’s individual risk of having a positive COVID test, based on the symptoms that they present with, and their exposure. All of it pulls data that is routinely captured in the context of a clinical visit. We built it all on the back end, where at the end of that visit, the physician would get a line or number next to the patient’s name in their screen that says, for each patient, “This person has a X% risk of being positive.”
What that would look like for a physician seeing a patient in the clinic, wondering if they should test them or not, and right now, with how limited testing is, they would have that show up in that visit, telling them if this patient is high-risk or not. From a patient’s end, they could be sitting at home. They go to the risk calculator and put in their symptoms. It gives them their individual risk, how likely are they to be sick.
This precision medicine at the level of routine clinical care, using data that is abstracted from the electronic health record, is another way of integrating research.
The third way that we’re doing to help with that integration is to use our prediction modeling to stratify patients by level of risk, again in the context of COVID, for different types of clinical trials that they may qualify for. Trials that are designed based on certain elements of effect size to demonstrate benefit: it helps them to know, going into the trial, the likelihood of achieving the outcome. It’s helping us allocate patients to different studies.
How can we connect patients who might benefit the most with that research?
I think it takes teams. We have tremendous work happening, but the challenge is that traditionally, it’s all happening in silos.
There are very smart informatics people who can talk a lot about the technical challenges, the technical pieces, but they’re talking to each other. You have researchers who are working on their own. You have clinicians working on their own. Patient advocacy groups working on their own.
My approach to it has been that nobody can do it on their own. We would all get so much more done if we work on this together. Once you agree on the “Why,” that patients are first and that helping them is our priority, then everything else becomes secondary, and then putting those teams together becomes easier when it’s done with a vision, with a goal.
With COVID, we started off putting together this registry; a huge endeavor, but everyone signed on. Within just two days, we had a protocol, we had a list of variables, we had an IRB approved, and it’s grown so much since then. We now have 13 different research work streams working within this registry. We have more than 200 ongoing clinical research projects on COVID, using that registry data.
Once we had that going, that gave us the population view. Then we created an interventional research team, and those were the people who organized all of the clinical trials that we have going. We are coordinating with them on the identification of subjects for their trials. We shared our data with our basic scientists who are doing the artificial intelligence work on host genomics, viral genomics and looking at potential drugs for repurposing and potential mechanistic understandings of comorbidities and how they link to COVID.
There’s been a lot of give-and-take between the two of us. Some drugs that we’ve identified, we’ve included in our registry to query, were based on preliminary work that they did. In our clinical research with the registry, we did find that those drugs are correlated with lower risk of catching COVID or getting worse with it. So then we communicated that knowledge to the interventional group and now they’re testing those drugs in their clinical trials.
Throughout all of this, we made sure to include our Office of Patient Experience, our Office of Caregiver Experience: people who look at our studies and research from the eyes of the patient. We have patient representation in our Institutional Review Board. We have patient representation in our data and specimen management committees.
It takes a village; no one can do it in isolation.
"The ultimate goal is to have this research facilitating clinical care, on the spot, as the patient is getting their care."
As more and more data becomes available, what are areas of caution or consideration?
The two big ones I think are privacy and agency. It’s a patient’s right to have their data, their information, be protected. It’s their right to decide what it can be used for.
We talk often about having these guardrails in research to protect our patients. But when you really think about it, only a small, small fraction of our data is what’s being used in research.
All of our lives, otherwise, are on our phones and out there for anyone to see. There are no guardrails on that. It’s out there, and we don’t really talk about it, but when it comes to research, we’re very strict, as we should be, to make sure things are done right. However, we cannot be putting research in a prison cell when data is running wild outside. There needs to be some alignment there. That takes conversations that require us, as researchers, to go outside of our comfort zone of science, and start talking policy and government.
The other challenge I see is that our focus on “Big Data” may be outweighing a much-needed focus on “Clean Data.” We are assuming that anything that’s big is good, and it may not be. If we have big data, but it’s all junk, that’s not going to help us. So spending as much time on figuring out how we clean what we are getting, and how we make it usable, is critical.
To learn more about Clinical Research as a Care Option, visit CRAACOevent.com.