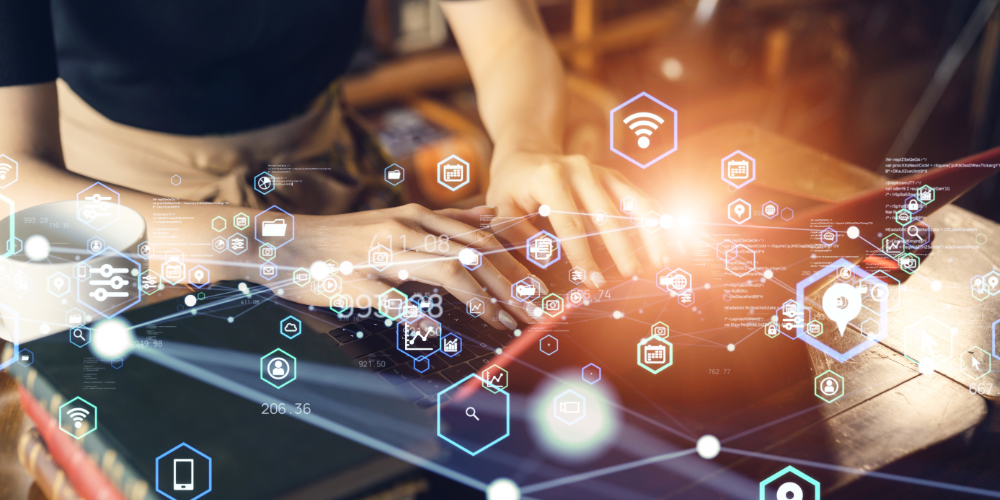
When you say sponsors should think of their clinical protocols as “data”, what do you mean?
For a lot of non-data-scientists, the word “data” typically conjures up images of numbers and spreadsheets. As a result, it can be hard to think of other things as “data” – such as written documents or notes, for example. But in reality, they are data, just unstructured and often underutilized.
Clinical protocols are a great example of this. Traditionally, a clinical protocol lives in a Word document. It is the way that we’ve historically had to represent, share, and store a protocol. But if you’ve ever put together, or even just read, one of these protocol documents, you’ll know that they’re full of so much rich information. It outlines exactly what the trial is designed to test, how it’s meant to be run, down to the most minute details. All of that is, at its core, data.
The problem we’re pointing out is that when all of that rich data is stored in Word or PDFs, it’s effectively locked away, unstructured, and impossible to use in any sort of automated way, whether that’s to make more data-driven decisions about trial design, or automate downstream processes for greater efficiency. When you start to think about protocols as data, you start to imagine all of the possibilities for how you could harness that data, all of the opportunities currently being left on the table.
How can this data be utilized for protocol design decision-making?
When you think about your protocol as “data” in its own right, you start to be able to make really data-driven decisions about the protocol as it’s being designed. Clinical trials have only become more complex over time, and overly complex trials are associated with poorer outcomes on trial performance metrics, such as recruitment, retention, or required amendments.
But if you’re not thinking of your protocol as data, it’s really hard to objectively quantify the complexity of your protocol. In our experience, it can be a real lightbulb moment once you start to be able to quantify complexity, and make decisions based on that data.
"When you start to think about protocols as data, you start to imagine all of the possibilities for how you could harness that data."
What have the results been, when implemented?
We ran an exercise in partnership with Merck, where study teams used Faro’s Study Designer across trials in six therapeutic areas to structure the protocol data and critically evaluate sources of complexity. The teams ultimately found over $130 million in potential cost reductions, and over 160,000 in potential patient-hours that could be saved by making specific changes to the protocols. Ultimately, they adopted changes that saved over $65 million and over 72,000 hours of patients’ time, which would not have been possible without a way to quantify the complexity of these studies.
In another case, we helped another customer identify an area of significant patient burden in their PK requirements, which would have almost certainly required an amendment later if they hadn’t identified it early and been able to discuss it with the regulatory agency. As it is, they were able to come up with a new approach that was much easier on the patient, while still meeting regulatory requirements. So, there’s very clearly a real immediate value to thinking about your protocol as data, and structuring it as such.
What about other, downstream applications of treating protocols as “data”?
For starters, a lot of our customers have started to migrate their historical protocols out of Word and into our Study Designer, and are using the data to evaluate their own performance improvements over time. That’s something that was really hard to do before, and something that is only going to be easier moving forward, as they adopt this new way of designing protocols in a digital-native way.
There’s also a very clear impact to downstream automation. Actually, back in July of this year, there was a great editorial on the DPHARM site all about the opportunities for automation in clinical trial operations, and how sponsors can take advantage of them. One thing that the contributors mentioned was the potential for automation to reduce or eliminate a lot of tedious, manual work that currently adds a significant amount to the overall clinical trial timeline.
Designing clinical protocols in a digital- and data-native way is one major way to unlock downstream efficiencies, because it creates a single source of truth for the trial. For instance, if you have a source-of-truth digital definition of your trial, you can use that to automate a lot of your EDC setup, potentially reducing your EDC build time by up to 50%. We’ve been able to do this with some of our customers already, and we’ll be excited to share more of those case studies in the near future.
There’s been a lot of interest in adopting AI technology in this space in the past year – how does “protocol as data” enable that?
It’s essential, to put it simply. Nearly every company in our space is currently trying to figure out how to fit AI into their business processes, so as not to be left behind. But many people underappreciate the absolutely critical part that data plays in making any insights or efficiencies from AI technologies possible. We expect that over the next few years, millions of dollars and hours are going to be spent organizing and structuring data in order to make it usable by new tools, in order to get any value out of it. That’s where we see Faro fitting in – our platform enables you to structure the data inherent in your protocol from the very beginning, so you can actually use it for AI-powered applications, and more importantly, get good results.
"When you have this depth of context, that’s when you really get great results. So it’s really important to make sure you’re setting yourself up to capture this level of depth and precision, and you’re laying the groundwork for all the possibilities this could enable in the future."
What is an example of where this platform fits into AI-powered applications?
Let’s take the specific case of generative AI for medical writing. There is a lot of excitement, rightfully, about the potential to leverage LLMs to significantly reduce the amount of time it takes to author the many necessary clinical and regulatory documents related to a clinical trial. The trouble is, as an industry we’ve found that general purpose LLMs aren’t inherently well-suited to the complexities of medical writing, and even if they were, you have to make your internal data accessible to these models to be able to actually generate any useful content.
Specifically to that last point, when you structure your clinical protocol in a Study Designer like what we’ve built, all of the detailed data associated with the protocol is now available for use by a domain-specific generative AI model. We’ve found that because we are able to help sponsors represent even the most complex Schedule of Activities (SoA) in our platform, we can generate Section 8 of the FDA’s M11 template (Trial Assessments and Procedures) in about 30 seconds. That’s really powerful, and not something that you could easily do if the SoA wasn’t represented as structured data for an LLM to use.
What would you advise sponsors to keep in mind, to really get the most value out of this approach?
Not all data is created equal. In that last example I shared, we talked about how having a digital representation of the SoA enables you to use generative AI really well. But you actually need a lot more than just a digitally-available SoA table – you need all the depth and precision behind it, to give the model the necessary context to produce generated content that’s really high-quality and indistinguishable from a human writer. For every assessment or procedure in that SoA, you need the detail that goes with it. So a chemistry panel isn’t just “chem panel”, it’s Chem-14 vs. Chem-20, it contains these specific panel members, it’s serum vs. plasma, it’s going to a local lab vs. a central lab, and it’s related to the objectives of the study in this particular way.
When you have this depth of context, that’s when you really get great results. So it’s really important to make sure you’re setting yourself up to capture this level of depth and precision, and you’re laying the groundwork for all the possibilities this could enable in the future. Coming back to that July DPHARM editorial we mentioned before, there was a great quote in that article from Taras Carpiac and Sheryl Jacobs at Amgen: "To take even better advantage of what automation has to offer, sponsors must make the upfront investment in data standards and underlying technical infrastructure." We believe this is absolutely essential, and now is a great time to do it.