Aitia’s CEO Wants You to Use AI to Do More than Designing Drugs Against Known Validated Targets
AI in the pharma R&D world isn’t just about designing better drugs against known drug targets, according to Aitia CEO Colin Hill. Mr Hill discusses how powerful AI can be in understanding novel human causal biology, creating novel drugs, and enhancing patient selection in clinical trials.
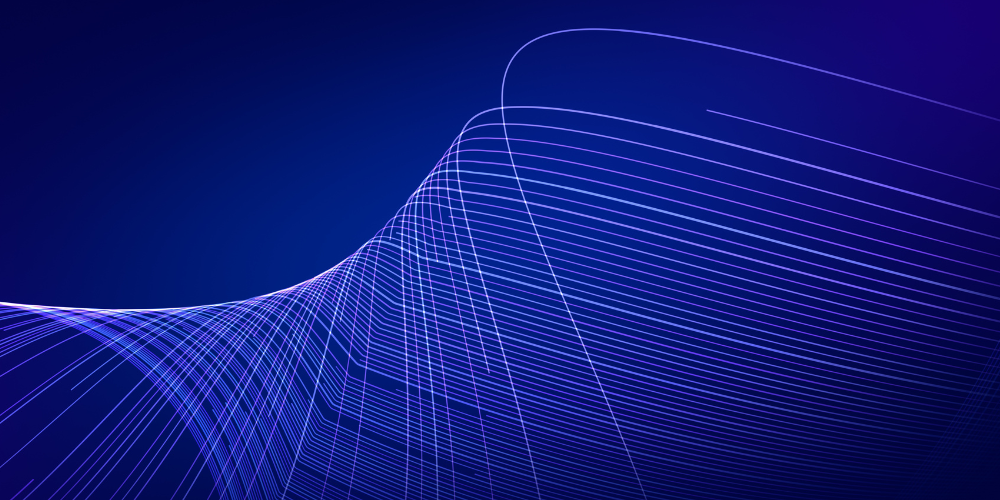
Where do you see AI having the biggest impact in R&D?
AI is starting to have its biggest impact in R&D in unraveling the unknown circuitry of human disease at the genetic and molecular level in order to discover truly novel drug targets causally linked to clinical endpoints. It’s also impacting the discovery of biomarkers, both prognostic markers of disease progression, as well as markers of drug response to better design clinical trials.
This is different from the low-hanging fruit the industry's been mainly focused on, which is AI to better design small molecules against known validated targets. That is causing a pileup around a small number of validated targets and leaving the rest of the genome somewhat untouched. AI being applied to better designing drugs is nice, but it’s not the transformative power from the convergence of genomics/multi-omics with supercomputing and new forms of AI, including causal AI.
In drug discovery, how does AI help achieve what would be otherwise impossible?
The complexity of human disease at a molecular and genetic level is enormous. It’s not just the number of components, genes or proteins, etc. It’s the way they interact and the number of these interactions becomes astronomically large. That goes beyond what the human brain and experts can fathom, especially when dealing with multiple layers of genetic, genomic, and proteomic data tied to clinical endpoints.
That's the kind of AI that excites me. AI in the pharma R&D world means the opportunity to go for the moonshot: unraveling novel human biology, creating novel drugs, chemical and biological drugs against those targets, and better designing clinical trials.
"AI being applied to better designing drugs is nice, but it’s not the transformative power from the convergence of genomics/multi-omics with supercomputing."
How do companies take advantage of that level of AI?
One, you need leadership that's scientifically deep enough, but has business acumen and some level of real influence and power within an organization. In some organizations, AI leaders are buried under functions such as IT or informatics. I think that's where the senior leadership gets confused as to what it is and are focused on just cost-cutting and efficiency gains.
Two, obtaining the right kind of data. This data doesn't have to be generated internally, but has to be data from humans. The paradigm of “in vitro to in vivo to clinical” doesn’t make sense when it comes to data and AI. We finally have the chance to start with data straight from human tissue and create discoveries from that tissue versus trying to make discoveries from cell lines or stem cells or organoids. Translatability from those systems to humans is probably the biggest single reason for the very high and very persistent clinical trial failure rate.
What is the hesitancy with moving forward with more AI models?
People are still tied to animal models and wanting to see preclinical data. Especially in neurodegenerative disorders, where we're focused, that approach doesn't make a lot of sense. It's hard for the industry to break away from what's been done for so many years and decades. Also, the venture capital industry wants ways to de-risk drug candidate programs before committing millions of dollars in clinical trials. But the relative weighting of evidence between human sources versus preclinical sources has to be rethought dramatically.
"AI in the pharma R&D world means the opportunity to go for the moonshot"
Are digital twins a suitable substitute for organoid or animal models?
A digital twin is a substitute, but it can also work hand-in-hand with those preclinical systems. We announced a partnership with Charles River Laboratories, and one very important part of the collaboration is that we're creating Gemini Digital Twins of PDX mice for a new level of translatability between that preclinical system and humans.
Computer models of those systems prescreen compounds before they're run through actual animal studies, and then the Gemini Digital Twins give this other level of translatability because now we can really mechanistically understand what the drugs are doing in those PDX systems, and connect that directly to humans.
Where do you see concerns around AI in R&D?
I have concerns that AI will get overhyped and the backlash will be to stop putting money into it. We’ve seen that cycle happen, with genomics, with the Internet itself. My other concern is about freeing up deidentified patient data. There is a reason for privacy laws both in Europe and the US, but they end up preventing the sharing of data necessary to actually generate insights that will lead to life-saving drugs. There needs to be a balance, and the risk-reward equation is tilted a little bit too much to the risk side.
I have zero fears in the world of pharma R&D that other industries have – such as the world of the consumer Internet where ChatGPT and large language models are spreading misinformation and worse. Those are real fears, but our industry is in a very different place. We don't have anything close to that level of data and our upside is much bigger and more important.
"We're in the early innings of seeing truly novel human causal biology come from these data sets in AI."
What do you see for the next steps with AI in R&D?
We're in the early innings of seeing truly novel human causal biology come from these data sets in AI. I think the industry will move beyond its fascination with the newest AI trends from Silicon Valley and get serious about the actual scientific problems to be solved in our world.
Applications like our Gemini Digital Twins that come from our causal AI technology and multi-omic human data are now resulting in novel, therapeutic candidates moving towards the clinic. We have one program that we think will represent one of the first real examples of a clinical stage drug candidate truly derived from data in a hypothesis-free way. The first examples will prove an important principle: a new and more powerful kind of AI applied to human multi-omic data on a large enough scale starting to derive insights that could not have been discovered by humans in hundreds of years.