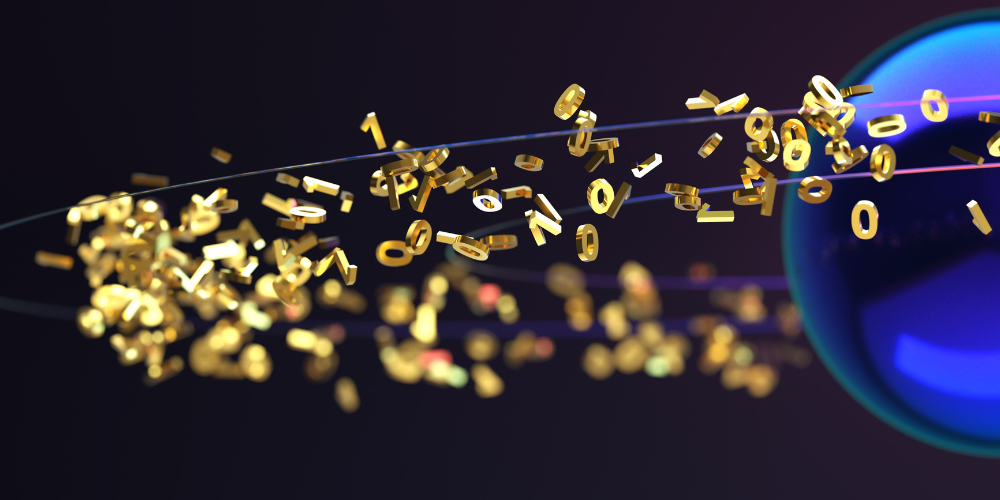
How have you seen the use of data science evolve to accelerate clinical research?
What I've seen over the past five years is an evolution towards taking data, combining it with knowledge, and pulling it together in order to make evidence-based decisions. Some examples that come to mind are real world data: leveraging claims data, medical records data, etc. This is taking the information that we have already collected for one purpose and it can be combined to be useful for other applications.
By looking at data in a broader, ecosystem way, we can start looking at what we know about the data, what biases we think the data has, and what its limitations are.
When you speak of the data ecosystem, what is the challenge of validating and defining data from different sources to then use them together or in new ways?
Different data sources have their own taxonomies, their own dictionaries and their own initial primary purposes of use. That applies also to internal data source systems. We cannot bring it all together if we don’t have a common map.
To bring it all together, if we don’t have a common map, we are lacking.
"Look at data as a company asset. If we think of data as an asset, how can we, in an appropriate, well-governed way, be able to reuse that data?"
What has the solution to that been?
We've seen a rise in the past few years of OHDSI, for example, and the OMOP common data model. But on the clinical side, when we submit clinical trial data, it's still in SDTM. There are still gaps. This vision of trying to have a data ecosystem where we can reuse that data requires that we think about how we map things and what our single source of truth is, as well as what the limitations of the data sources we’re bringing together are.
Have you seen movement in creating a single source of data “truth?”
The FAIR principles: findable, accessible, interoperable and reusable. If there's one thing that we can do, simply for organizations to know what data they have is a starting point.
If we can make it as easy as a Google search, to be able to say, “I'm looking for something.” You may already know exactly the data asset you're looking for, and the indication that you want to pursue, but you may have no idea in which data assets across the organization, you may find that information. The ability to just at least make it findable already supports that ultimate vision of a data ecosystem.
How do we encourage people to make data accessibility more of a priority?
Look at data as a company asset. If we think of data as an asset, how can we, in an appropriate, well-governed way, be able to reuse that data? That requires a different mindset to rethink how we can utilize this data in a way that is different from its initial purpose.
"Nowadays, it's so easy: you can just go in, you can do an analysis and you can come out and walk away. But we have to still be so careful; we cannot do this in the absence of thinking."
Where else could you see RWD or EHR data being incorporated to aid in decision-making?
Real world data is what we have collected for things, such as claims or medical records. When it comes to rare diseases and the ability to understand that patient journey, there might not even be a diagnosis code for that. So how do you know what that looks like? And how do you follow up a patient to understand the pain points that they're experiencing on their journey with their disease?
There might be even the opportunity to work with other organizations, where you might have some text that we as pharma perhaps shouldn't be seeing, but we can work with someone else to help get that from a semi-structured language to something more structured. That could allow us to understand “This patient was diagnosed with this condition. Let’s look back at what that journey was, where the pain points were and if we could have involved them in a study at this particular stage.” It gives you a chance to make things more patient-centric to our best ability.
When it comes to rare diseases, there may not even be a diagnosis code for that. But if we can use real-world evidence and EHR data, we can understand the patient's journey better: where the pain points were and at what stage we could have approached them with a trial.
Data became a huge priority during COVID; what did you learn from that?
Through the pandemic, I've marveled at the way we visualize COVID data. There have been some interesting ways that people have thought very creatively about how to visualize that kind of data. I hope we keep that as a data community, this interest in trying to think about and looking at data from slightly different perspectives and letting data help to tell the story. In the past, we’ve always stuck with a long report full of tables. And what we’re seeing more of now is a more dynamic way to interact with data. It may not be the best way to confirm, but it does open up the door to generate new hypotheses and new insights, that we can then appropriately validate in a regulated way. Down the road, I would love to see a data ecosystem across pharma, sharing data where appropriate and with the patient at the center. And that will benefit the entire community.
As we generate more and more data from various sources, what is your advice to make the best use of it?
I once read a quote from a statistician remembering the days when it cost money to run an analysis. You had to plan ahead, and you had to be sure about what you wanted to do. Nowadays, it's so easy: you can just go in, you can do an analysis and you can come out and walk away. But we have to still be so careful; we cannot do this in the absence of thinking.
I would love to see more of that same rigor, that same scientific approach and learning-fast culture be brought forward to the data science and healthcare intersection. That means that we are very thoughtful about what the source of the data is, what the strengths and limitations are, and what the appropriate ways we should be using data assets are.
And then where we can, where we think that risk is appropriate for the question we have at hand, it would be amazing to see us try different things, learn, generate new hypotheses and then have an environment in which we can also then validate those. What we see in publications is a lot of the positive findings; we don’t see a lot of the work that was done behind the scenes, experiments that maybe didn't go right. Those are as much learnings as anything else. We don't have a way where we can share that. That's where the data science community has a lot of opportunity to learn and grow together.