What Data Informatics Could Do For Your Clinical Research Study, with an Informatics Expert
Data’s full potential to expedite clinical research can't be realized without standardizing data capture or sharing. Meredith Zozus, PhD, CCDM, talks about the impact of informatics upon clinical research. She is Professor and Division Chief, Clinical Operations and Director, Clinical Informatics, at University of Texas Health San Antonio.
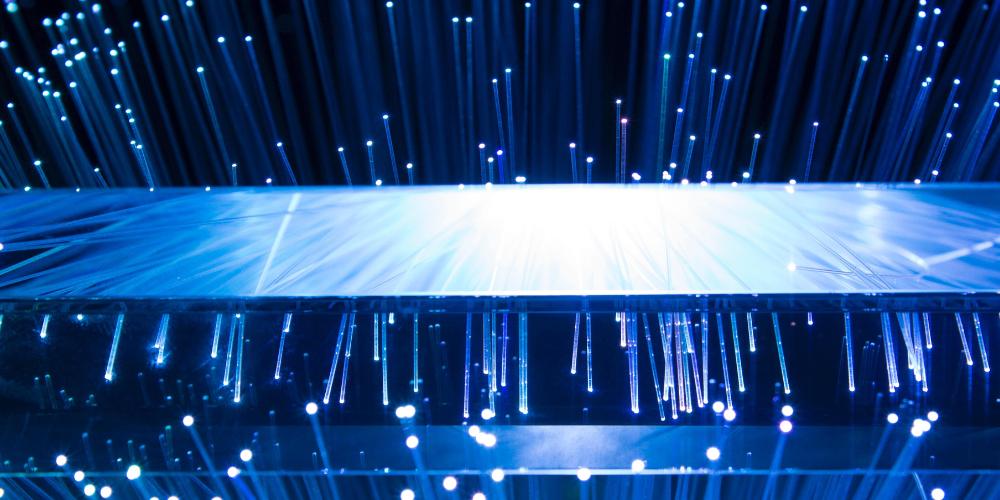
What would disruptive innovation look like in your field?
Let me take the innovative angle first. For innovation, it’s really all about doing things that you couldn’t do before, or as an informaticist, enabling people to do things that they couldn’t do before through new or better information. Whether it’s being able to monitor a trial differently, whether it’s being able to manage a study differently, whether it’s being able to interact with patients or providers differently, or collecting data that you couldn’t collect before. All of those things mean innovation.
Right now, the chain of custody of data is broken. Because people abstract data manually out of medical records, there’s a break in the traceability between the EHR and the study database. But if we can get the data electronically and directly from the EHR, we can also get the audit trail electronically and directly from the EHR, and establish that complete chain of traceability in and along with the data that we receive for studies. This traceability in the data all the way back to the EHR source doesn’t exist now for the FDA.
And when you think about disruptive technology, if you think about some of the work that’s been done in grassroots clinical trials, or direct-to-consumer clinical trials, that doesn’t have to be so different from prospective research or site-based research. What if we can add in that interaction directly with patients to do things like assuring the quality of the data on an ongoing basis? That’s disruptive; it’s less expensive than split samples and independent ways of capturing the data.
The evidence that we have shows that patient self-reported data is capable of detecting errors in electronic health record data. People previously thought that detecting errors in EHR data was not possible. Where the EHR is the source (the original recording), once the patient visit has ended, you can’t go back and recapture the source. This use of direct interactions with patients and the technology and the approaches to do so could be very disruptive from a standpoint of assuring the quality of EHR data used in clinical studies. Similarly, other options exist for identification and correction of errors in the source. We are also pursuing those. Enabling identification and correction of errors in EHR data enables the use of EHR data by regulators. We’d like to see this possibility for marketing authorization, not just post-marketing, safety surveillance, etc.
"Right now, the chain of custody of data is broken. If we can get the data electronically and directly from the EHR, we can establish that complete chain of traceability."
Where would you like to see us in five years, in terms of how we utilize the EHR?
There’s so much that needs to change with respect to that. People think it’s a panacea, but for decades, people have acknowledged that the quality of the data in the EHR is lacking.
The first thing we need is better standardization of EHR data. The Health Level Seven (HL7) FHIR® standards are progress towards doing this but are relatively new for use in research. The HL7 Fast Healthcare Interoperability Resources (FHIR®) standards identify data elements and those can be mapped to studies. The mapping is like a thread that pulls the right data values through from the EHR to the right place in the study database. Both the National Institutes of Health (NIH; through NOT-OD-19-122) and the regulated industry through formation of the HL7 VULCAN accelerator are encouraging the use of FHIR® standards in clinical research. But also, there needs to be interventions within healthcare facilities to increase the quality of the EHR data. That’ll help some for research but will always be targeted to healthcare needs, i.e., the data that the facilities need, care about and use, and will never comprehensively address all of the research data.
The other thing as far as use of EHR data in years to come: right now, we do well-controlled clinical trials for marketing authorization. That’s a pretty slow, expensive process. That’s also a pretty limited process, from the standpoint of subgroup analysis, disparities and heterogeneity of treatment effect. Our well-controlled clinical trials, in a lot of cases, don’t do a great job at foreshadowing effectiveness in the real world.
I would love to see us, with respect to use of EHR data in five or 10 years, decreasing the dependence somewhat on well-controlled clinical trials. Yes, we need them. However, let’s speed that up; let’s get into the wild sooner, in a provisional way, where we’re getting data on every patient. That way, we don’t just have, say, 3000 patients that have been on a drug for a year or two, we have 30,000 patients or more that have been on that drug for a year or two. It allows us to get data earlier, and detect safety signals, disparities and heterogeneity that we usually are not able to detect in the way that we approach drug development today.
Can you describe your work with machine learning in ICU settings? How can we be utilizing AI/ML to greater effect?
The more immediate promise for AI and ML is on the clinical side, toward precision medicine and predictive analytics, and dropping that down to the front end of the electronic health record, for the physician to say, “This option may be better than that one for my particular patient.”
And I’m at a health system; so we’re seeing those uses being called for immediately. We’re seeing physicians that want to develop better models, rather than clunky risk scores where they have to type in 20 pieces of information. But we need much more scalable models. At the top 60 medical centers, everybody’s got data in one or more of the standard common data models. And crosswalks now exist between them through work at FDA and NIH and through the CD2H and N3C initiatives. It’s relatively easy to machine over those for training and to apply them in health systems that already use the common data models. The FHIR® standards can also be used in that way and are available now on most major EHRs in the US, so ML approaches are becoming more accessible. But It’ll be a while before community hospitals are used to doing that.
In clinical trials, it’s some of the same challenges. It’s the challenge of doing ML and AI at scale and building it into information flows in our drug development processes. On the clinical side, we can do it more easily at scale by getting it into the EHR and bumping up the predictive analytics up to the front-end clinical decision support features available in most major EHR systems.
In clinical trials, you’ve got these custom data processing pipelines that are based on EDC systems and maybe 3-5 more other data sources in a study. Right now, we don’t have the software that leverages the standards, and enables us to do things like machine learning and AI at scale. There are people that are trying it within large data processing operations, and they’re having to build it from scratch. It’s going to take a few years for the software and the standards to catch up to doing that in clinical trials. But the premise of signal detection, decision support and improving human performance in whatever they’re doing for the clinical trial is a worthy pursuit.
"I would love to see decreasing the dependence somewhat on well-controlled clinical trials. Let’s get into the wild sooner, in a provisional way, where we’re getting data on every patient."
What would the better use of informatics in clinical research look like for you in the more immediate future?
There are multiple opportunities. Jules Mitchel has published something on this, as has Wolfgang Summa. These publications show that people aren’t looking at the data when it comes in through EDC as soon as they could be. They’re not setting expectations that the data to be entered the same or next day, and they’re not looking at it the same or next day; they’re waiting weeks. And so, they’re losing that opportunity to intervene on processes that adversely impact the study. That is one immediate use of informatics, with information systems we have today to start looking for signals, reviewing, and using data more quickly.
For the other, I’m going to go back to direct EHR data acquisition. The largest error rate of all the data quality and data processing methods is the medical record abstraction process. And so, although you’re not going to save time on it, that error rate for medical record abstraction is an order of magnitude larger than other data processing error rates such as entry, record linkage, or coding. People don’t realize this, they don’t talk about this. When you look at just getting wrong data, that’s an order of magnitude higher, and it’s more variable. There’s this latent source of error (in the human factors sense) and variability in our underlying data from the manual medical record abstraction process, from human inconsistency in which data to select under a myriad of available scenarios. Then we look around and wonder why we get equivocal results, or why a trial fails to show efficacy.
Using facilitative technology for direct EHR data capture allows you to intervene in the cognitive process of the person abstracting the data. Maryam Garza’s work showed that in the analyzed study, the medical record abstraction error rate could be reduced by a maximum of about 65% if you can get that data electronically. So, is it saving money? Not really, especially not the first few times you go out in the field with it. But from a standpoint of tightening down the data, and getting that unwanted variability out of the data, it’s huge for companies. That’s where the real value is. Aside from that, the FDA finds value in having that unbroken chain of custody back to the EHR data source. And we can clean EHR data; in fact, we can probably do it better than is currently done in traditional clinical data management processes.
What are the areas in clinical research where we could be doing better to innovate?
Other industries have catapulted themselves forward with use of the Internet, online interactions and direct interactions. We’ve really stumbled in a lot of ways in the earlier direct-to-consumer trials and using that, in part because we haven’t used it appropriately. You can’t cut the sites out. You need the sites to recruit the patients, and you still have to cover the site costs for doing that. Queries over the local data warehouse may be useful, or a trial may not be able to be recruited without decision support implemented in the EHR so study coordinators and providers know before or during the encounter that a patient is potentially eligible. These cost money to program and sites have to have the clinical research informatics expertise. These opportunities to accelerate recruitment and enrollment are huge. There is a lot of untapped potential here for sponsors.
For more information on DPHARM: Disruptive Innovations to Modernize Clinical Research, visit DPHARMconference.com.