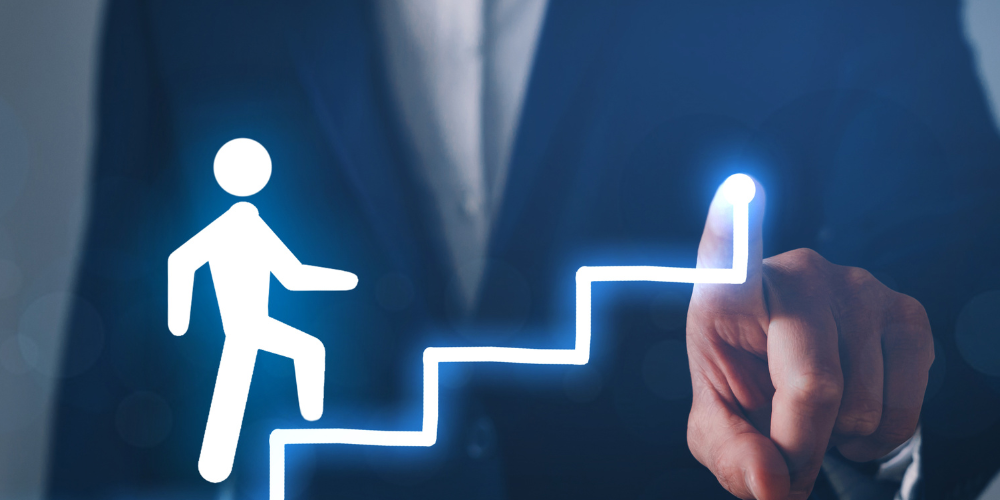
How are you applying innovation in clinical trials at Regeneron?
Innovation drives patient-centricity and should be objective-focused. Innovation should also be supported by a scalable process. Innovation is not chasing something just for innovation's sake. There are many innovations we choose not to explore, or we take a “watch and wait” approach because we don’t yet know whether the technology is mature enough, or if it’s truly a fit for the problem we are working to solve.
An example of this would be artificial intelligence. Many things, such as prediction capabilities, fit very well with what we do in operations where we can apply AI. However, other applications need to mature more, for example, the operating parameters of large language models, before being fit into industrialized use.
What are the main areas your team has been tasked with making more efficient?
My role in development innovation is specifically about finding ways to make our clinical trials faster and more efficient. One of the top areas of feedback we get from sites and investigators is how much work is needed to manually transcribe data from their systems into the study forms and data capture systems, even when they’re all computerized. My team is looking at how we can do less manually while maintaining or increasing results in our clinical research.
One example of this is how we can now move study data directly from electronic health records and eSource systems into our electronic data capture systems. This is a prime instance of an innovation that has matured enough in our opinion for utilization now that US requirements for EHR FHIR (Fast Healthcare Interoperability Resources) standards adoption finally “kicked in” in 2021-2022. The benefit of this is to not only lower the burden on research site personnel but also decrease querying and manual source data verification on the sponsor side. This may net potentially higher data quality that can be gathered more quickly.
"Innovation drives patient-centricity and should be objective-focused."
What is an example of how you’re innovating in a non-data field?
We also have the opportunity to use technology to improve culturally-specific ways of crafting messages. I’ve seen the impact of COVID-related behavioral economics work I collaborated on with the World Health Organization while I was at Google. There are similar approaches we can use for outreach to patients and clinical investigators. In the setting of required DE&I plans, it’s even more relevant. This is an innovation that relies on human behavior, psychology, and behavioral economics, rather than technology. Still, it uses data to drive what we think is the best approach – not opinions only.
How are you thinking about advancing to the next stage of modernizing clinical trials?
It’s about the cost of courage. To advance, we need to build up the courage to change on a larger scale. Our industry is notoriously risk-averse, but I would submit that many of us dare to try new and different things in our everyday lives. We need to have that same courage to believe we are capable of trying something different in clinical research. It’s difficult to do, but once you establish that courage, people realize they can do the work – and that’s critical for the next phase of modernization in clinical trials.
Where will AI be useful, in your opinion, for current tasks?
One category where AI will be useful is optimizing things that people can do but AI can also do well at scale. These include tasks that can be more easily validated because we can compare them to human performance. If we become better at articulating what we’re attaching AI to – text summarization, prediction, classification, etc – it becomes clearer as to whether or not it’s a fit.
"Ultimately, we will be able to do studies with fewer patients and in faster timelines if we truly understand the disease."
As engineer friends have taught me, think of a human doing an activity. Could someone really good at the activity do it better than anyone else? That indicates that machine learning-based AI might better fit that task.
Where will AI excel in ways that humans cannot?
Another category where the industry will benefit is machine learning-based AI activities that are impossible human tasks, such as prediction. But these tasks come with additional challenges: you must design an entire testing and validation paradigm once you believe the performance is optimal and error rates or other risks have been mitigated.
However, for biostatistics and real-world data – that have their origins in basic math – I’m far more confident that we can make the case for AI because you can trace the mathematical pathway, and use newer methods to optimize it even further. One of the advantages of deep learning is that it has a fundamentally different approach to looking at data and hidden relationships between independent variables, which is not what classical statistics was designed to solve.
What aspects of clinical research is AI mature enough right now to have a strong impact?
Right now, there is utility in understanding the trajectories of disease. We can use that in multiple domains, including enriching trials for patients who are more likely to show an effect earlier, or retrospectively to be able to include a much larger population and then refine the noise using AI models. Ultimately, we will be able to do studies with fewer patients and in faster timelines if we truly understand the disease.
However, there is further development needed in terms of regulatory acceptance of these types of approaches, to find ways to critically evaluate the methods that we would use in these cases. I would also caution against going all in on AI-assisted study designs. That suffers from a lack of clinical scientific input and is divorced from the deep understanding of study limitations. An example of this would be broadening inclusion/exclusion criteria that, if examined more closely, wouldn’t make sense to a clinician in the context of the study.
"We look to not only try to imitate what’s traditionally done, but also look at how new approaches can bring us closer, sooner to scientific answers."
You’ve worked inside and outside of pharma to advance and scale innovation. What is your best advice for securing buy-in for new innovation?
First, you need to be sure that you have a data-driven, logical argument behind it. Get away from innovation for innovation's sake, because you can only go so far with style. You need to bring the substance. Especially in larger organizations, change management is crucial; it’s more compelling to logically persuade someone using data. Regeneron has a very data-driven culture, which is similar to when I worked at Google. In both companies, there are deeply scientific technology and engineering folks who want to understand the problem in tremendous depth, as well as how the innovation you’re proposing has been tested.
Second, it requires testing all of your assumptions and inviting critiques and further hypothesis testing. This approach makes any argument for an innovation stronger, and it’s the most effective at cultivating innovation long term.
Where do you look for inspiration for innovation?
In some ways, pharma is similar to the entertainment industry; we write a script (or a protocol), there is a massive amount of planning, and there are many different steps to go through to get to the end product (an approved product). But some of the breakthroughs in Hollywood have been possible because of the newer technologies available. For example, using building-sized LED display walls to be able to film on a sound stage rather than having to go on-location.
There are remarkable parallels in how we are rethinking the notion of where different activities need to happen in traditional clinical trials versus technology-enabled trials. Even if some innovations are not directly translatable to our work we can test underlying basic principles in similar ways. At Regeneron, we look to not only try to imitate what’s traditionally done, but also look at how new approaches can bring us closer, sooner to scientific answers.