Archive Highlight: Turning Data Science from Abstract to Reality, with Janssen’s Chief Data Science Officer
Dr Najat Khan shares her experience developing the foundation – the people, the processes and the change management – required for fully utilizing data to enhance clinical development. Dr Khan is Chief Data Science Officer and Global Head of Strategy and Operations for Janssen Research and Development.
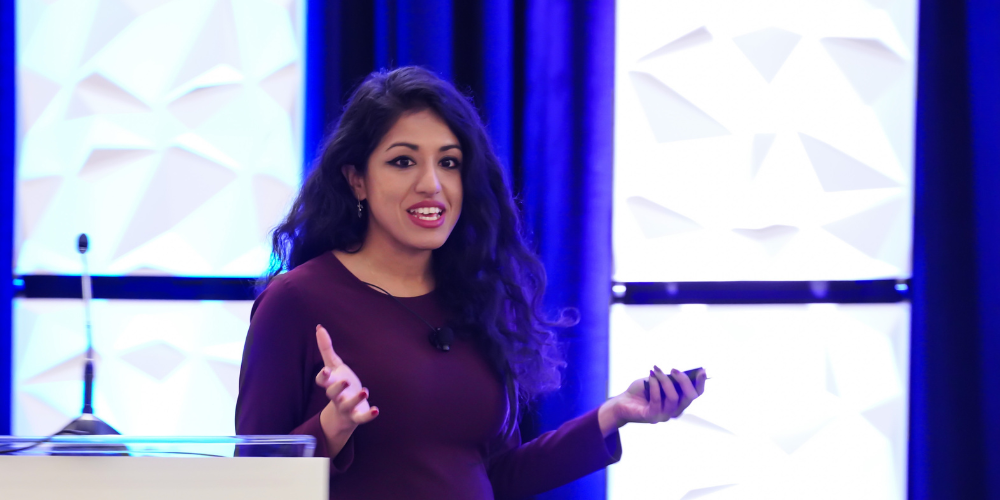
How is Janssen using data science to vastly improve its R&D process?
At Janssen, we’re leveraging data science – AI, machine learning, real world evidence and digital health – to truly transform how we discover and develop medicines and vaccines. It spans the entire gamut of R&D – from the earliest stages of discovery through to clinical trials. We focus on coupling deep knowledge of our pipeline with insights derived from data science to determine where we have the biggest opportunities for impact across our therapeutic areas – and how we can maximize our chances of developing potentially game-changing new medicines, more efficiently than ever before.
How many projects are applying data science to their development?
We have over 100 data science projects covering about 90% of our pipeline. It is totally upending how we approach research and development. Before, people would read scientific literature, and say, “This is what the disease is, and this is how we should design and run our trial.” Now, every time before we set our sights on a target or get ready to launch a human trial, our teams partner shoulder-to-shoulder with data scientists.
Data scientists look to see what is happening in the real world to patients: what medicines they are on, if they are effective, who is responding to the treatment and if we can detect those patients earlier. We are asking how we can use AI/ML to find where the patients are to decide where we set up our trials. That’s the fundamental core: you’re changing the design and development of a therapeutic with a singular focus on helping the patients we serve.
"Often, I’ll see folks go after a cool new algorithm that was developed or a shiny new platform. None of that matters unless you’re addressing something meaningful."
Can you share a specific example of how data science is being utilized?
One example is the rare disease pulmonary arterial hypertension. There are medicines that can slow down disease progression, but patients are often diagnosed too late to maximize the value of these treatments. We used real world data – 100 million anonymized patients in the US – to learn that patients were getting misdiagnosed, which we knew, but by an average of four years. But we also learned that the one of the first tests that patients generally get after the onset of symptoms is an ECG.
We can use deep learning to look at the nuanced changes in a patient’s ECG, to be able to flag that this person could have this disease. This is a rare disease; not every primary care physician actually thinks about it, and the symptoms, such as shortness of breath, could be attributed to so many other things. If we can use data science to help identify patients earlier, we can make full use of the medicines available to significantly improve patient outcomes.
How did Janssen use data science in its COVID-19 trials to get a vaccination approved?
We developed a machine learning model with MIT and were able to predict, with 90% accuracy, where the hotspots would be, on a county level – globally – four months in advance. We predicted going to the South, to the Midwest, to South Africa. And those places were exactly where the hotspots were four months later. This helped to shave six-to-eight weeks off our development timeline, enabling our vaccine to begin reaching people quicker at a time where every moment counted.
We were also able to recruit a highly diverse trial, including African-American and Latinx patients. We had over-indexed going into regions where there was more diversity, and over-indexed on regions expected to have less social compliance, such as mask-wearing. The behavioral component is not something usually used in these models. We had to trust a model that had never been used before, and to use it for one of our most-watched trials ever. And to actually then have the kind of impact we did, is huge.
"We had to ask, “What are the big questions we need to answer, and therefore, what are the datasets we need to pull together to do that?”"
How do you embed data science capabilities into trial planning?
What COVID did was provide the momentum. To be able to have a proof point that you can do things differently opens up the possibility of what can be achieved when data science is applied.
As I mentioned earlier, we were able to get to 100+ use cases across 90% of the pipeline in a year and a half because of our core foundations that are built for scale. We have a platform called Med.ai that connects our preclinical, clinical and real world data. All the models that are being developed are in one place. It’s important because you can reproduce things done for one project in other areas; it’s no longer a one-off. That platform has been a huge technical feat that has allowed us to be able to scale and do it sustainably.
When you first took over the department, what was the first step to connect all of Janssen’s data?
The first thing I wanted to do when taking on the role was to create a platform to glean predictive insights from a patient journey. We built it in a test-and-learn way, but with the outcomes in mind. We had to ask, “What are the big questions we need to answer, and therefore, what are the datasets we need to pull together to do that?” This is not a data lake. The data has to be high-quality, traceable and linked. A lot of work went into that, but we were able to do it.
What was the change management required once the technical side had been achieved?
That was almost as challenging, because datasets in general are in silos. But when you actually pull resources together, you can answer questions that benefit the entirety of what we’re doing. You cannot do data science at scale without having a foundation like that.
There are also the people required to make it happen. The data scientists on my team are bilingual in data science and medical science. The person who developed Med.ai is a physician technologist. She understands omics data and hospital data very well, and can collaborate with our scientists and operations leads. This is a different way of working, one that creates bridges. And you can create much tighter bridges if you speak a similar language.
What is an example of how data science fundamentally changed something about the trial design process?
We have a vaccine program for invasive E. coli. It is a challenging disease that generally impacts older people. We knew that there must be other factors so we looked at 100 million patient lives, from a completely unbiased approach and no hypothesis. We just let the data speak to us.
We were able to validate that age is a high correlator, but we also learned that there were actually 4-5 novel risk factors that had never been considered before. When we talked to clinicians, they said, “That makes sense, but I had never thought about it.”
"The data scientists on my team are bilingual in data science and medical science. This is a different way of working, one that creates bridges."
What was so powerful about that example for you?
We actually ended up incorporating those novel factors into our trial design. To see that being incorporated into our ways of working was one of the first example to me of the power of data science – and of our leadership’s commitment to leveraging that power to transform R&D.
Also, one of the hardest things is when people don’t pick the right questions. The “What” is so important. Often, I’ll see folks go after a cool new algorithm that was developed or a shiny new platform. None of that matters unless you’re addressing something meaningful.
What part of the discovery process would you like to see fundamentally shifted by data science in the next three years?
One is in how we design and conduct our trials. The ideal state for me would be that before we even start thinking about a disease, we apply AI and machine learning toward all of the data that’s available to define that disease. That’s not happening as much, but it will be more in the next couple of years. This is in the drug discovery space: AI- driven drug discovery, so that you go after the right protein, or target, that’s causing the disease.
And number two, where there is some great work already happening, is being able to predict what the right molecule is. You want to have safety, specificity and high affinity. If you get those two things right upstream, then the probability of success for everything you do downstream improves. When we systematically do that to have novel targets and novel insights, we will be able to develop treatments for diseases that were previously untreatable. That is still early stage, and the next 3-4 years are going to be critical, and we’re doing a lot in that space as well.
For more information on DPHARM: Disruptive Innovations to Modernize Clinical Research, visit DPHARMconference.com. To see Dr Khan speaking on enabling an end-to-end digital transformation in clinical research, click here.